Table of Contents
In an era dominated by the integration of AI systems into our everyday routines, ensuring their safety and security has become an imperative task. Recent strides in AI research have ushered in groundbreaking techniques aimed at eliminating potentially perilous intelligence from these systems.
In this article, we will explore the significance of securing AI systems, exploring breakthroughs in purging hazardous data, and examining real-time illustrations that underscore the critical importance of this development.
The Importance of Securing AI Systems
Securing AI systems is paramount in today’s technological landscape. As it continues to permeate various sectors, including healthcare and finance, its potential for enhancing efficiency and decision-making is undeniable.
However, this very reliance on this also exposes vulnerabilities that malicious actors can exploit. Without proper safeguards, these systems are susceptible to manipulation, leading to potential harm and chaos.
Therefore, ensuring the security of AI systems is imperative to safeguard against threats and mitigate the risks associated with their deployment.
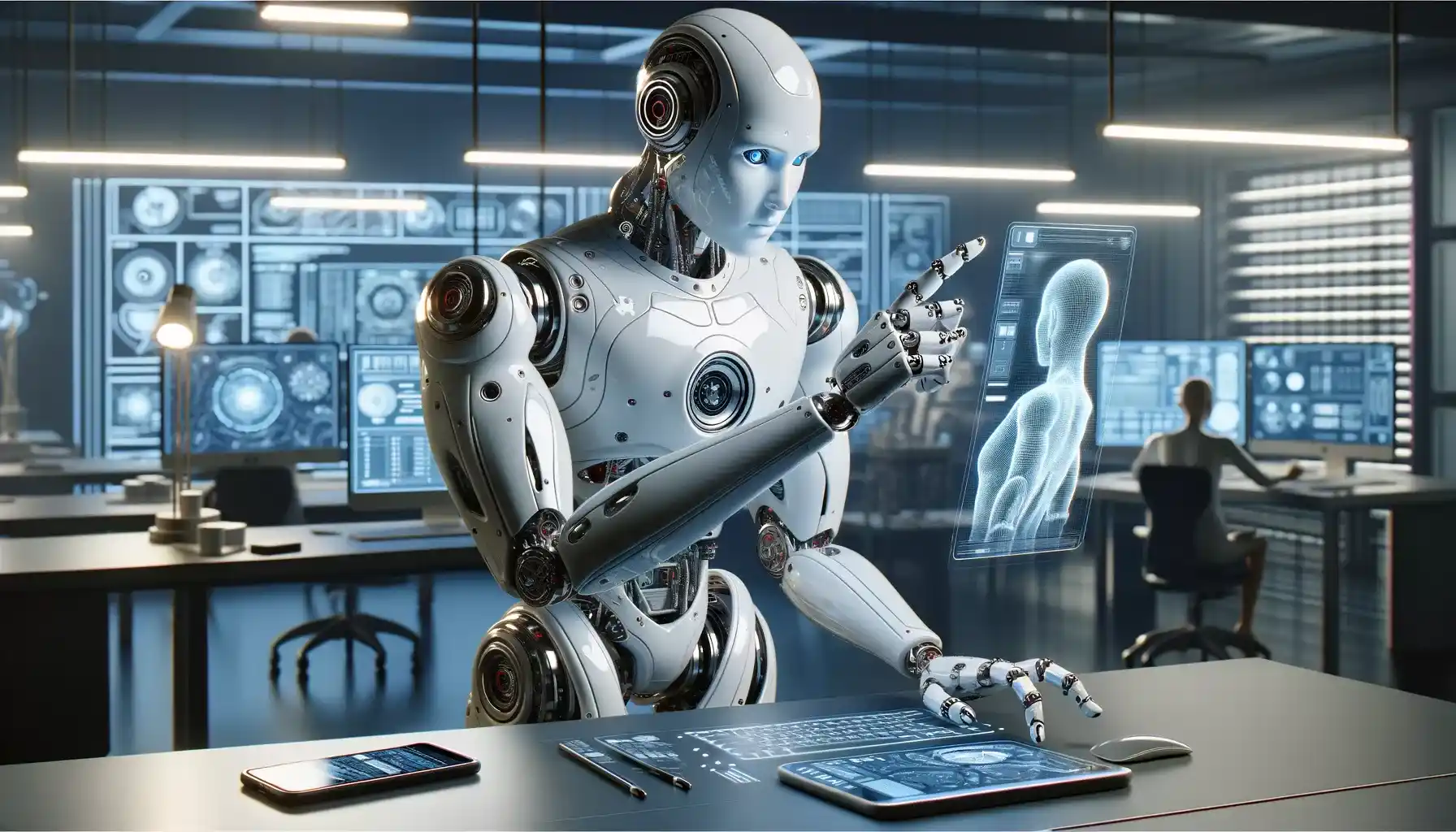
Moreover, as it also evolves in complexity and capability, the need for robust security measures becomes even more pressing. These systems are tasked with critical functions, such as automating tasks and analyzing vast datasets, making them lucrative targets for cyberattacks and breaches.
The consequences of compromised AI systems can be severe, ranging from financial losses to compromising sensitive information or even endangering lives in sectors like healthcare.
Hence, prioritizing the security of AI systems is essential to foster trust, protect user data, and uphold the integrity of the systems that power our modern world.
Breakthrough Purges Perilous Intel
In recent years, researchers have prioritized addressing the potential risks associated with AI systems, particularly concerning the dissemination of sensitive or harmful information. One notable advancement involves the development of sophisticated algorithms designed to identify and mitigate these risks effectively.
For instance, companies like Google have implemented advanced techniques to detect and remove biased or discriminatory content from their AI-powered algorithms. By employing these algorithms, AI systems can operate more safely and ethically, reducing the likelihood of inadvertently perpetuating harmful biases.
Furthermore, the evolution of AI scrubbing techniques has become essential in various sectors, including healthcare. Medical AI systems, for instance, must adhere to stringent ethical standards to ensure patient privacy and safety. Researchers have devised methods to systematically review and sanitize medical data utilized by AI models, ensuring that confidential patient information remains secure. This advancement not only enhances the trustworthiness of AI-driven healthcare solutions but also safeguards patient confidentiality in increasingly digitized medical environments.
Moreover, the continuous refinement of AI scrubbing methodologies holds promise for addressing emerging challenges in areas such as autonomous vehicles.
As these vehicles rely on AI systems to make split-second decisions, it is imperative to eliminate any potentially hazardous or unreliable data that could compromise safety. Engineers are leveraging advanced algorithms to thoroughly vet and sanitize training datasets, reducing the likelihood of AI systems making erroneous decisions on the road.
By integrating robust scrubbing techniques, AI-powered vehicles can operate with greater reliability and mitigate risks associated with accidents or malfunctions.
Real-Time Illustrations
1. Autonomous Vehicles
In the domain of AI systems, the development of self-driving cars poses unique challenges, notably the potential for these vehicles to inadvertently adopt risky driving behaviors. For instance, if an AI-powered car is trained on data that includes instances of aggressive or risky driving, there’s a risk it may mimic these behaviors on the road, compromising safety.
Real-time instances highlight instances where such behavior could manifest, such as a self-driving car making abrupt lane changes or overly aggressive maneuvers in traffic.
To address this issue, developers have introduced breakthrough techniques like purging, which allows for the identification and elimination of perilous intelligence within AI systems. By systematically identifying and removing instances of risky behavior from the training data, developers can ensure that self-driving cars prioritize safety and adhere strictly to traffic regulations.
Through the implementation of purging techniques, AI systems can be fine-tuned to operate reliably and responsibly on the roads, mitigating potential risks associated with learned behaviors.
2. Healthcare Diagnostics
In the realm of healthcare, AI systems play a pivotal role in medical diagnostics and treatment recommendations, leveraging vast datasets to aid clinicians in decision-making. However, the reliance on historical data poses inherent risks, as AI systems can inadvertently learn biased or inaccurate information, leading to erroneous diagnoses or treatment plans.
For instance, an AI system trained on historical data that disproportionately represents certain demographics may exhibit biases in diagnosis or treatment recommendations, potentially exacerbating healthcare disparities.
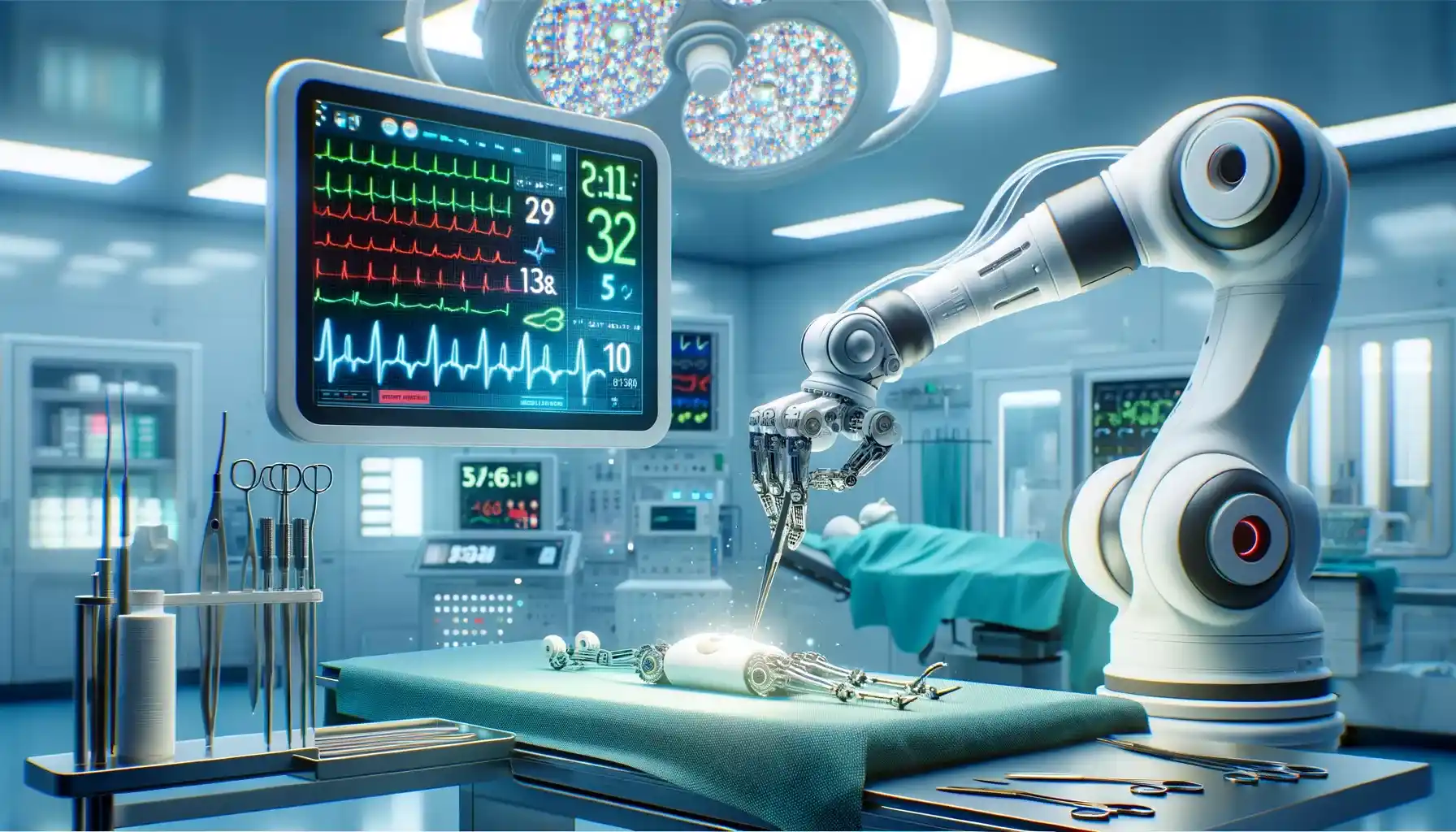
To mitigate this risk, ongoing efforts focus on purging perilous intel from AI models, employing techniques such as data preprocessing and algorithmic auditing to identify and address biases. By ensuring that AI systems are trained on diverse and representative datasets, healthcare practitioners can enhance the accuracy and reliability of diagnostic and treatment recommendations, ultimately improving patient outcomes.
In a real-world instance, a study conducted by researchers at Stanford University revealed biases in an AI system used for skin cancer detection. The system demonstrated higher accuracy in diagnosing skin lesions on lighter skin tones compared to darker skin tones, reflecting underlying biases in the training data.
To rectify this, researchers employed techniques to balance the dataset and mitigate bias, enhancing the system’s performance across diverse skin tones. Such efforts underscore the importance of actively purging biased or inaccurate information from healthcare AI systems to uphold accuracy and fairness in medical diagnostics and treatment recommendations, thereby fostering equitable healthcare delivery for all patients.
3. Financial Fraud Detection
It play a crucial role in detecting financial fraud, but they are susceptible to compromise if not properly secured. Breakthrough purging techniques offer a solution to this challenge by safeguarding AI models against malicious manipulation and ensuring the integrity of the data they are trained on.
For instance, by employing techniques like differential privacy, financial institutions can protect sensitive data used to train AI systems from being exploited by malicious actors. Additionally, continuous monitoring and updating of AI algorithms can help identify and address potential vulnerabilities before they are exploited. Integrating fraud risk management practices further enhances the system’s robustness.
In a real-world scenario, a leading bank implemented advanced purging techniques to fortify its AI-based fraud detection system. By regularly purging fraudulent data from its training sets and employing adversarial training methods, the bank enhanced its AI system’s resilience against evolving fraud tactics.
As a result, the bank significantly reduced false positives and improved its ability to detect sophisticated fraudulent activities, safeguarding its customers’ financial assets. Such proactive measures demonstrate the importance of integrating breakthrough purging techniques into AI systems to bolster financial fraud detection capabilities and protect against potential threats.
Implications and Future Directions
The breakthrough technique, exemplified by the systematic purging of perilous intelligence from AI systems, represents a pivotal advancement in bolstering the security and reliability of such systems. For instance, through rigorous filtering processes, AI systems can eliminate vulnerabilities exploited by malicious actors, enhancing trust among users and stakeholders.
This development not only ensures current AI applications operate with heightened security but also catalyzes further research and innovation in AI security, laying the foundation for even more robust and resilient systems in the future.
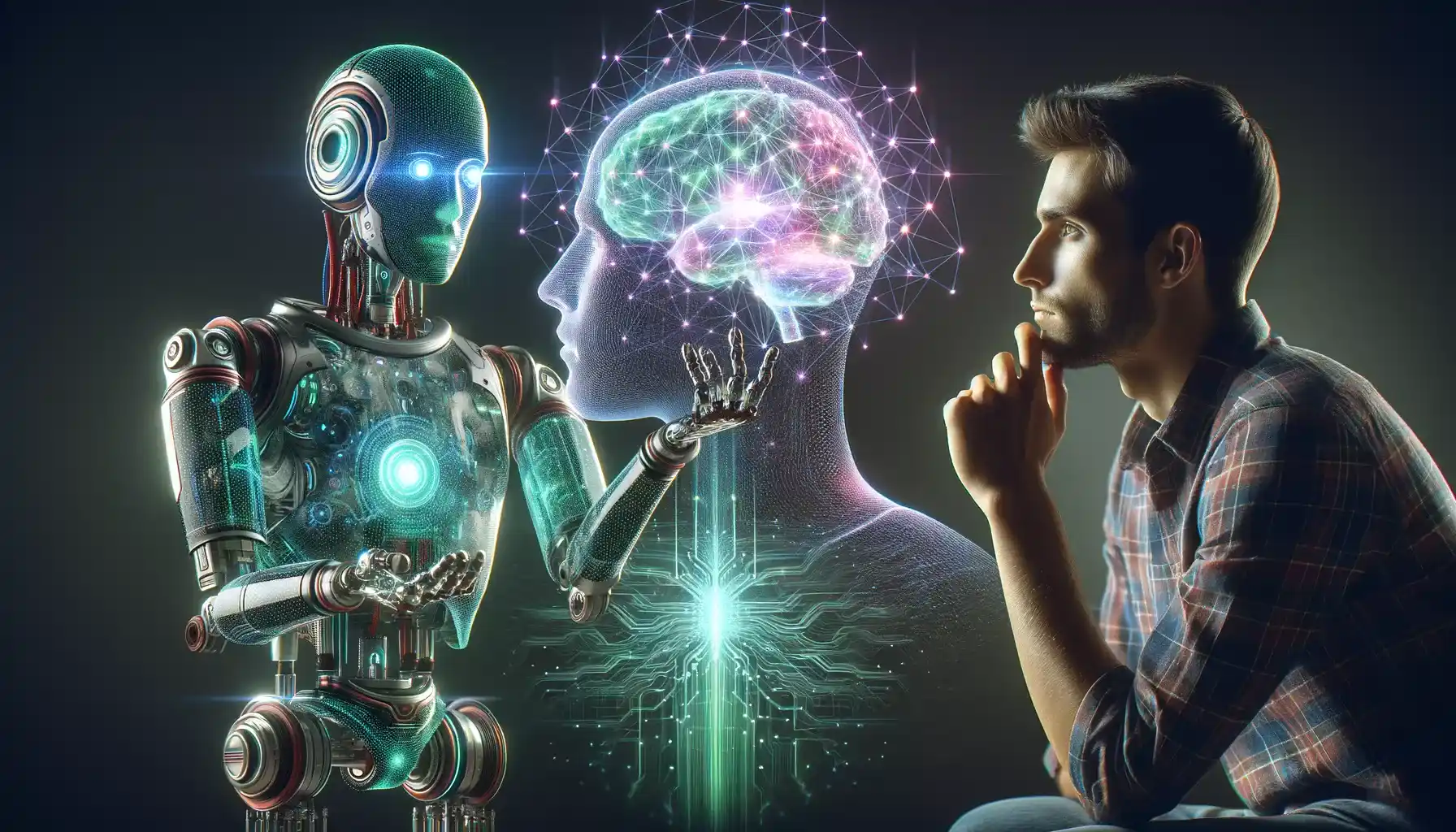
Conclusion
As AI systems continue to integrate into diverse societal domains, safeguarding their security and integrity is paramount. The breakthrough purging technique stands as a crucial advancement, enabling proactive measures to mitigate risks associated with potentially dangerous intelligence.
For instance, in autonomous vehicles, implementing this technique can ensure that AI algorithms consistently prioritize safety over other objectives, leading to enhanced reliability and trustworthiness in their operation. This proactive approach not only fosters a safer future but also instills confidence in the widespread adoption of AI systems across various sectors.