Table of Contents
The integration of AI and machine learning into battery management systems (BMS) is indeed revolutionizing the way we approach energy storage and utilization. This technology is particularly vital for enhancing the efficiency and lifespan of batteries, which are central components in renewable energy systems and electric vehicles (EVs).
AI and machine learning algorithms can analyze vast amounts of data from battery management systems operations, including temperature, voltage, current, and state of charge. By processing this data, these systems can make real-time decisions to optimize battery performance. This includes adjusting charging rates to prevent overheating, balancing cells to prolong lifespan, and even predicting future battery health and maintenance needs.
In the context of renewable energy, where energy sources like solar and wind are intermittent, AI-enhanced batteries can ensure a more reliable and steady power supply. They can intelligently store excess energy when production is high and release it when demand peaks.
For electric vehicles, AI-driven battery management systems can significantly improve range and battery life, two of the most critical concerns for EV users. By intelligently managing the charging and discharging cycles based on usage patterns and environmental conditions, AI can help maintain battery health over longer periods, reducing the need for EV replacement batteries.
Furthermore, as the world moves towards a more sustainable and eco-friendly energy infrastructure, the role of AI in battery management becomes increasingly crucial, paving the way for smarter, more efficient, and resilient energy systems.
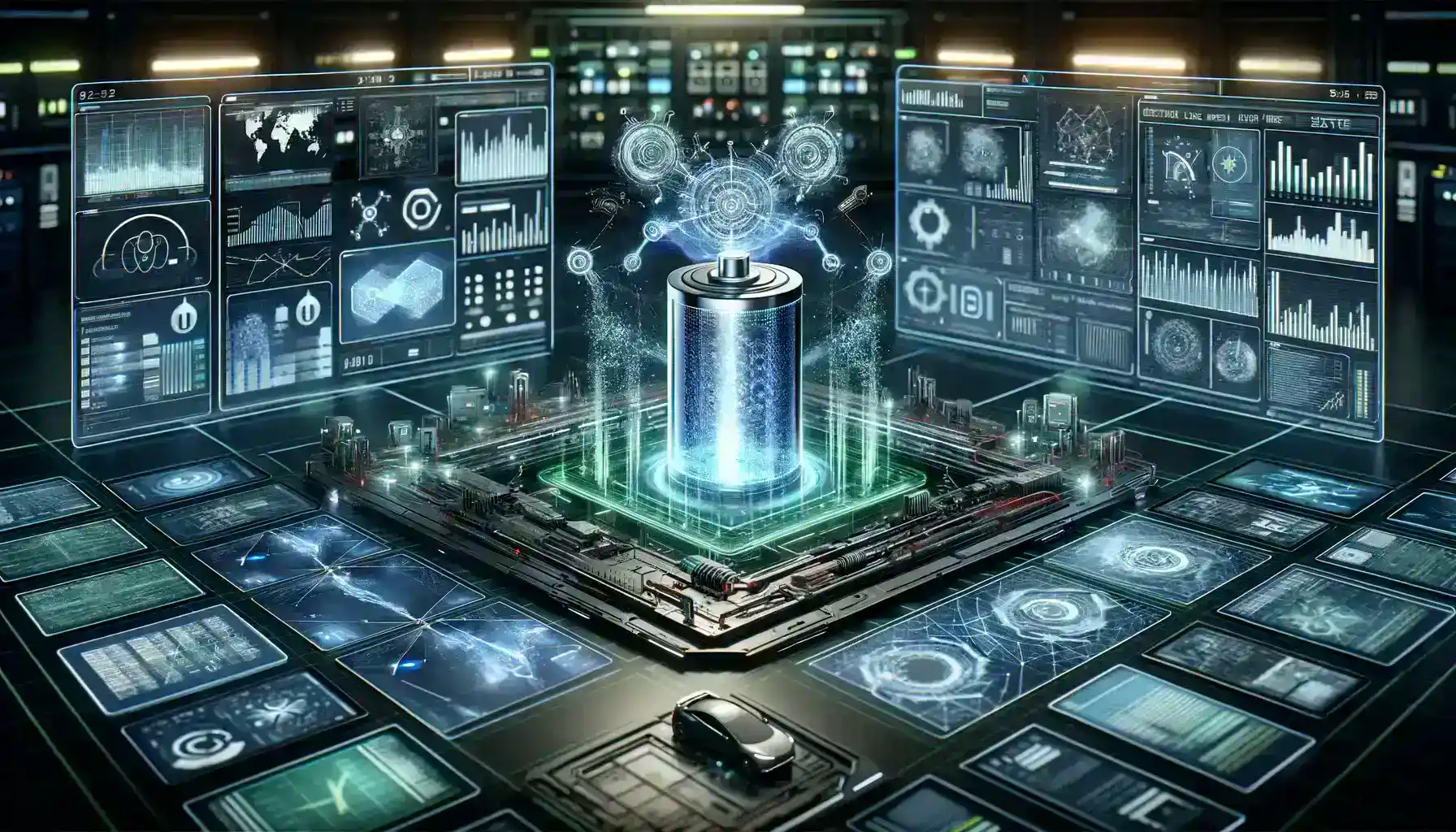
Enhancing Battery Performance: Leveraging Battery Management Systems
The capability of AI algorithms to accurately predict and optimize the charging and discharging cycles of batteries plays a vital role in not only extending the lifespan of the batteries but also in maximizing their efficiency.
In electric vehicles (EVs), where battery management system performance is directly linked to the vehicle’s range and reliability, this application of AI is particularly significant. Tesla has been at the forefront of employing machine learning techniques to improve the performance of their EV batteries. These AI-driven systems continuously analyze data from the vehicle’s operation, including battery temperature, usage patterns, and charging habits. By understanding these patterns, Tesla’s AI can optimize charging strategies to reduce battery strain, prevent overcharging, and evenly distribute charge among cells, thereby enhancing the overall health and longevity of the battery.
This optimized charging and discharging not only extends the life of the battery management system but also ensures that the vehicle operates at peak efficiency. This means longer ranges on a single charge and a reduced likelihood of battery degradation over time, which is a key concern for EV owners.
Moreover, this technology is not just limited to EVs. It’s also being applied in various other sectors where battery management systems play a critical role, such as in renewable energy storage systems, portable electronics, and grid storage solutions. The ability of AI to adapt and learn from real-time data makes it an invaluable tool in the quest for more durable and reliable energy storage solutions.
Predictive Maintenance:
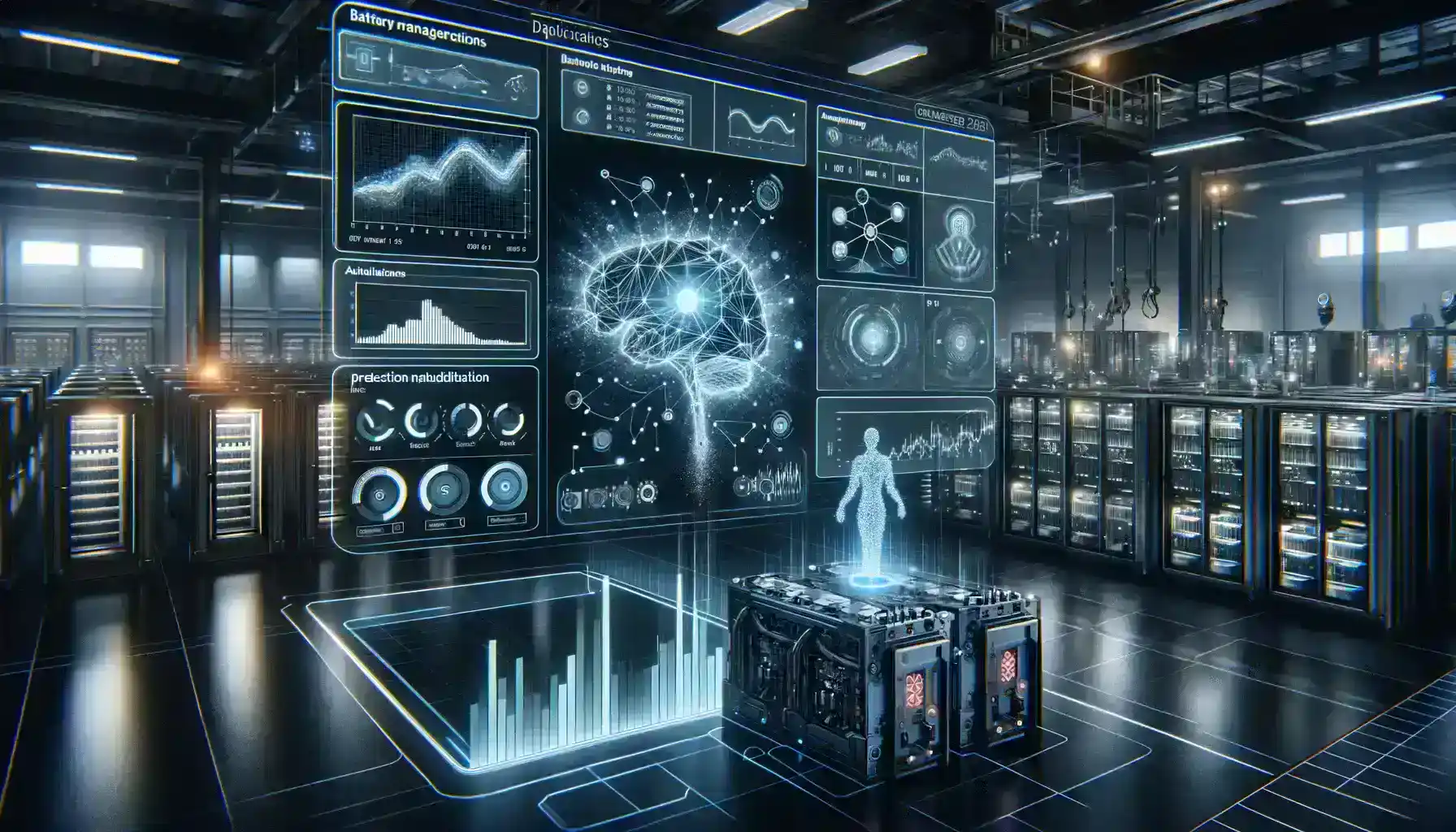
Machine learning models are adept at analyzing patterns within large datasets. In the context of a battery management system, these models process data from various sensors monitoring the battery’s state, such as voltage, current, temperature, and charge cycles. By learning from this data, the models can identify trends and anomalies that may indicate potential issues or future failures.
This capability allows for predictive maintenance of battery management systems, which is a proactive approach as opposed to the traditional reactive methods. Instead of waiting for a battery management system to fail or show signs of degradation, predictive maintenance can alert operators to potential issues before they become critical. This early detection is key in minimizing downtimes and preventing costly interruptions, especially in industrial settings where battery management system reliability is crucial.
Siemens implements an AI-driven battery management system that can predict battery health and performance issues. These systems continuously analyze battery data and use machine learning algorithms to detect signs of wear or impending failure. By doing so, they can provide alerts and recommendations for maintenance before the battery’s performance is significantly impacted.
Predictive maintenance not only extends the operational lifespan of battery management systems but also enhances the safety and efficiency of the entire system in which they are used. It’s a proactive strategy that saves time, reduces costs, and improves overall operational efficiency. As battery technology continues to evolve, the role of AI and machine learning in predictive maintenance for battery management systems is expected to become increasingly vital, particularly in sectors where reliability and uptime are critical.
Safety and Reliability:
The aspect of safety and reliability in battery management systems, enhanced through AI, is a critical development, especially in the realm of consumer electronics.
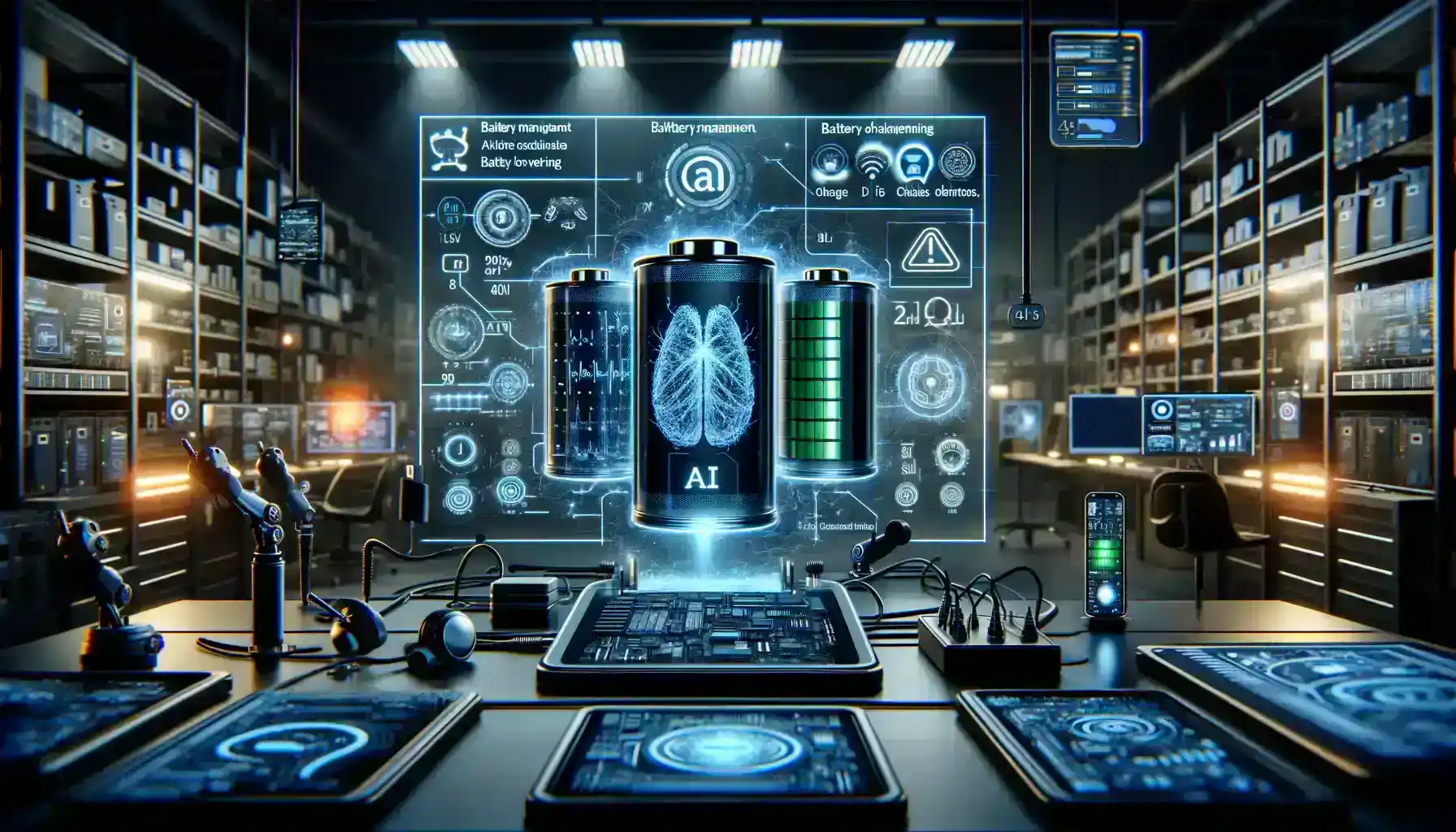
AI plays a pivotal role in ensuring battery safety by continuously monitoring and analyzing various parameters such as temperature, voltage, charging rates, and usage patterns. One of the key concerns with batteries, particularly lithium-ion batteries used in consumer electronics, is overheating, which can lead to dangerous situations like fires or explosions.
By employing AI algorithms, potential hazards like overheating can be detected much earlier. These systems can predict when a battery is likely to overheat based on usage patterns and environmental conditions. When such risks are identified, AI-driven systems can take proactive measures, such as adjusting charging speeds, reducing power consumption, or even alerting the user to potential safety risks.
Apple is a notable instance in this regard. They have incorporated AI-driven management systems in their devices to monitor battery health actively. This not only enhances the safety of the device by preventing issues like overheating but also helps in maintaining optimal battery performance over the device’s lifespan. For instance, based on the analysis of the battery’s condition and usage patterns, the system can optimize charging routines to reduce wear and tear on the battery, thereby prolonging its life and maintaining its efficiency.
The integration of AI in battery safety is not just limited to preventing overheating but also extends to other aspects like improving the overall reliability and performance of the battery management system. This is particularly important as the world increasingly relies on battery-powered devices, from smartphones to electric vehicles. The advancement of AI in battery safety and reliability is a key step towards more sustainable and dependable energy storage solutions.
Optimizing Charging Infrastructure:
AI also plays a critical role in managing electric vehicle charging stations. It helps in balancing the grid load and reducing energy costs. Smart charging stations use AI to adjust charging rates based on grid demand, enhancing efficiency.
Demand-Responsive Charging: AI algorithms can dynamically adjust charging rates based on the current demand on the electrical grid. During peak times, when the grid is under heavy load, these systems can slow down charging to alleviate stress on the grid. Conversely, during off-peak times, they can speed up charging. This demand-responsive approach helps in maintaining grid stability and can also benefit consumers through lower electricity rates during off-peak hours.
Load Balancing: In areas with multiple charging stations, AI can distribute power efficiently among different vehicles to maximize charging speed and minimize wait times. This load balancing is crucial in public or semi-public charging locations, like parking garages or shopping centers, where there can be significant variance in EV charging demand.
Predictive Analysis for Infrastructure Planning: AI can also aid in predicting future charging needs based on trends in EV usage, helping city planners and private companies make informed decisions about where to expand charging infrastructure. This predictive planning is essential for ensuring that the growth of EV charging networks keeps pace with the increasing number of EVs on the road.
Integrating Renewable Energy Sources: AI can also be used to optimize the integration of renewable energy sources, like solar or wind, into EV charging infrastructure. By predicting when renewable energy production will be highest, AI systems can schedule EV charging during these times, further reducing the carbon footprint of EVs.
User Experience Enhancement: AI-driven systems can offer personalized charging experiences for EV drivers, such as recommending the best time and location for charging based on their travel patterns and preferences.
Through these capabilities, AI not only enhances the efficiency of EV charging stations but also plays a crucial role in the broader energy ecosystem, supporting the transition to cleaner energy sources and more sustainable transportation options.
Energy Storage and Grid Integration:
The integration of AI in energy storage and grid management is a key development in modern energy systems, especially in the context of incorporating renewable energy sources.
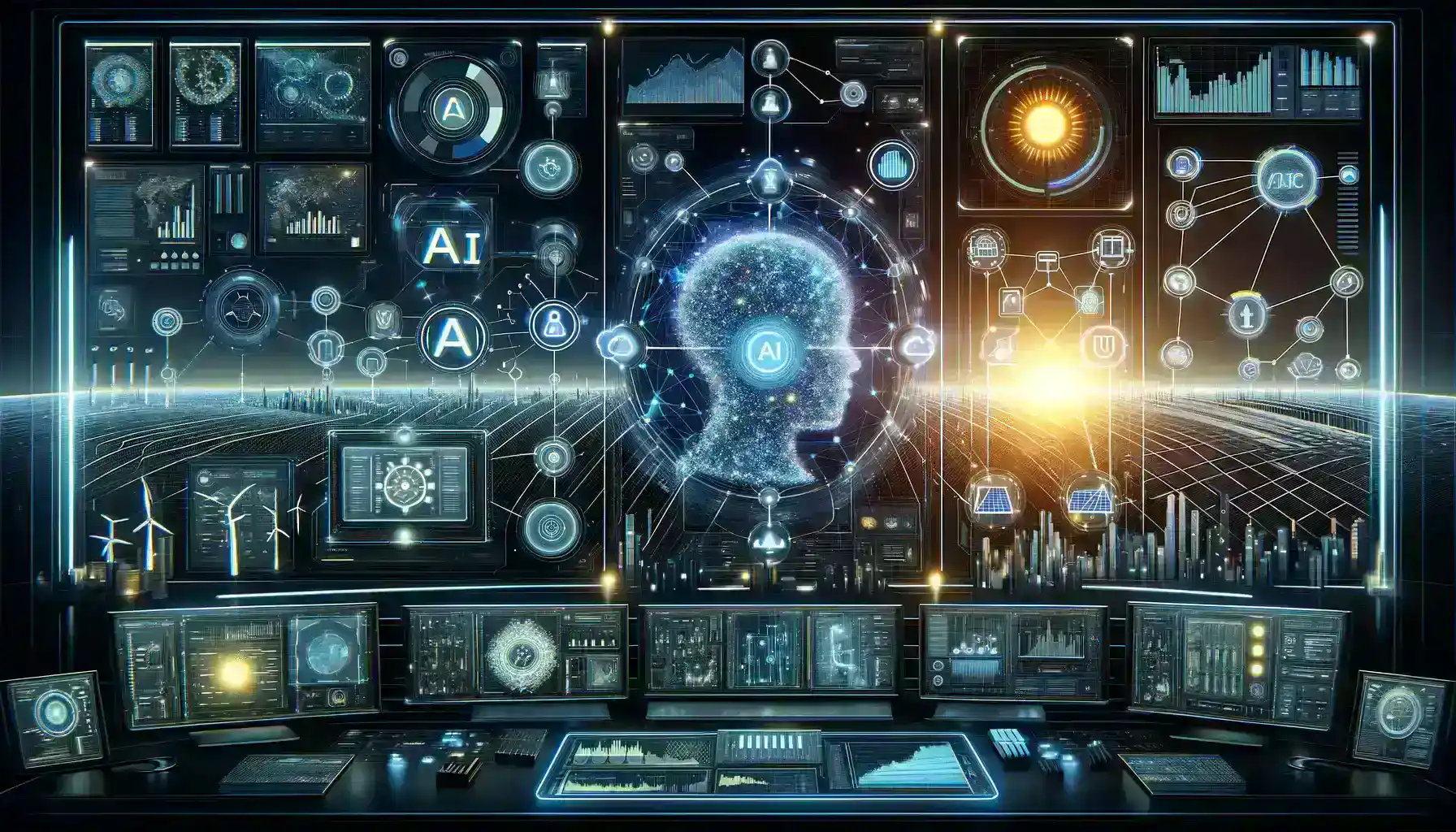
Optimizing Battery Storage: AI algorithms are instrumental in optimizing how energy is stored in large-scale battery systems. These algorithms can predict energy demand patterns and adjust how much energy is stored or released from batteries. This optimization is crucial in situations where energy generation from renewable sources like solar and wind is variable and intermittent.
Balancing Supply and Demand: AI systems can analyze vast amounts of data, including weather forecasts, energy usage patterns, and grid conditions, to predict energy demand. By accurately forecasting these demands, AI enables battery storage systems to release energy during peak times when supply from renewable sources might be low, and store energy when there is an excess. This balancing act is essential for maintaining the reliability and stability of the power grid.
Grid Stabilization: With the fluctuating nature of renewable energy, grid stability can be a challenge. AI-driven battery storage systems can respond rapidly to changes in energy supply and demand, helping to stabilize the grid. This rapid response is crucial in preventing power outages and ensuring a consistent energy supply.
Case Study – Enel X, a global company focusing on energy solutions, is a prime example of an AI application in energy storage and grid integration. Enel X uses AI to manage their large-scale battery storage systems, ensuring that energy is stored efficiently and released precisely when needed. This not only helps in balancing the grid but also maximizes the utilization of renewable energy sources.
Economic Efficiency and Environmental Impact: By optimizing battery storage and grid integration, AI contributes to both economic efficiency and environmental sustainability. Efficient energy storage and use reduce the need for fossil fuel-based energy during peak demands, leading to lower greenhouse gas emissions and a smaller carbon footprint.
Conclusion:
AI and machine learning have indeed revolutionized the field of battery management systems, marking a significant leap in how we approach energy storage and utilization. These technologies are not just incremental improvements but are pivotal in enhancing the efficiency, safety, and longevity of battery systems. This revolution is particularly important in our current era, where the shift towards renewable energy sources and electric vehicles is accelerating.