Table of Contents
Deep learning, a subset of machine learning inspired by the human brain’s neural networks, has witnessed a remarkable evolution in recent years. This article explores seven game-changing breakthroughs in deep learning, with a focus on the pivotal role of Deep Neural Networks (DNNs) in reshaping the landscape of artificial intelligence.
As deep learning continued to advance, it gave rise to a series of game-changing breakthroughs. These breakthroughs expanded the horizons of what AI systems could achieve and revolutionized various industries. Let’s explore seven of these transformative advancements, all of which owe their success to the foundational role of Deep Neural Networks:
1. The Inception of DNNs in Deep Learning
The inception of deep learning marked a pivotal moment in the field of artificial intelligence. Deep Neural Networks (DNNs) were at the core of this innovation, paving the way for the development of more complex and layered neural networks. DNNs introduced the concept of hierarchical feature extraction, allowing machines to automatically learn intricate patterns from data.
The inception of Deep Neural Networks in deep learning stands as a testament to human ingenuity and the relentless pursuit of creating intelligent machines. This foundational breakthrough laid the groundwork for a cascade of innovations that have redefined industries, research, and everyday life.
They achieved breakthroughs in image classification, speech recognition, and language translation, surpassing the performance of traditional machine-learning approaches. It meant that DNNs could excel in a wide array of tasks, from image recognition to natural language understanding and beyond.
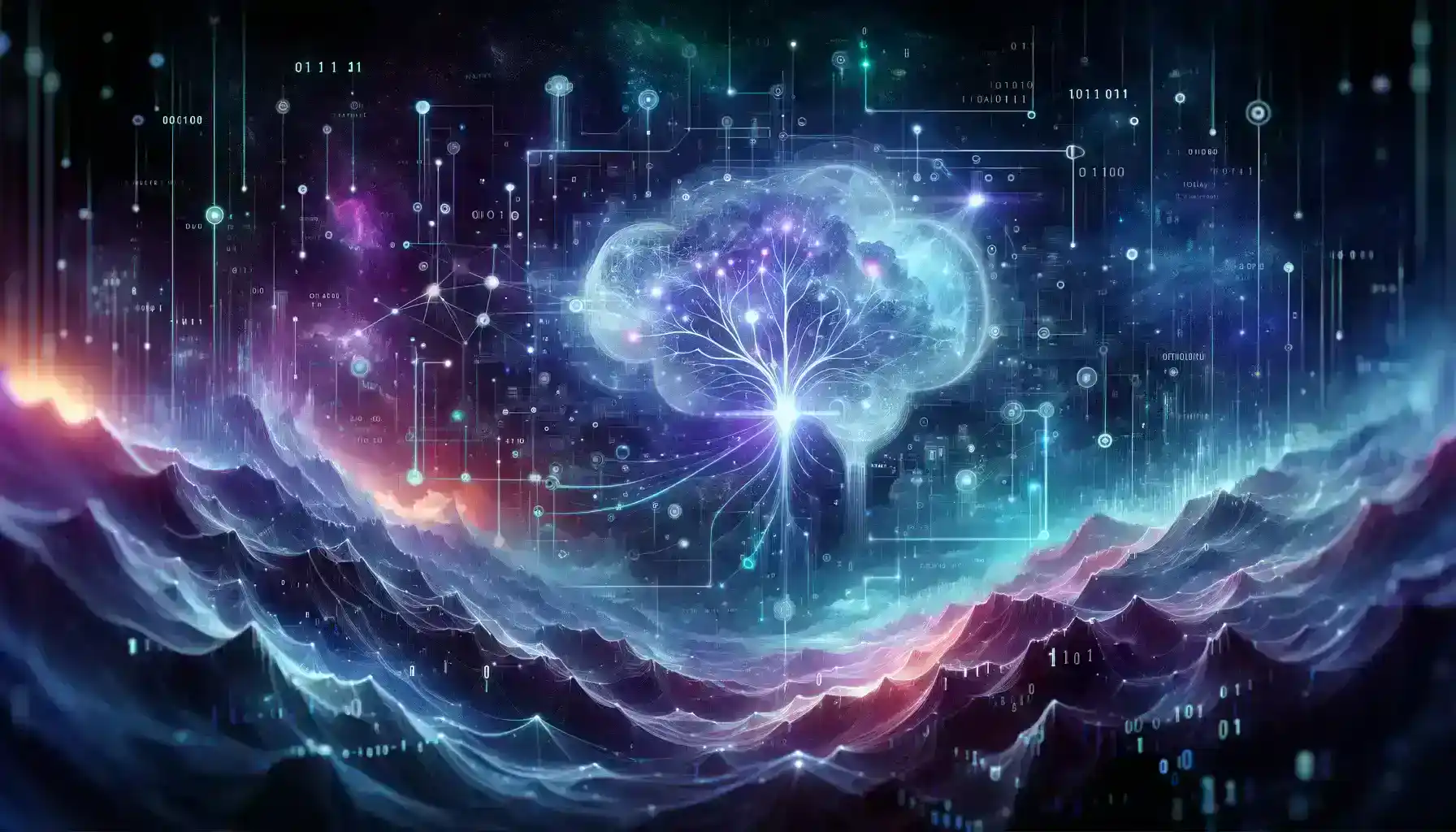
2. The Ascendance of CNNs in Deep Neural Networks
Convolutional Neural Networks (CNNs), a subset of Deep Neural Networks (DNNs), have become synonymous with image processing and computer vision. CNNs utilize specialized layers called convolutional layers, which enable them to automatically detect and understand visual features within images.
These convolutional layers are designed to automatically detect and understand visual features within images, making CNNs exceptionally adept at “seeing” and interpreting visual data. Researchers are constantly exploring ways to improve the efficiency and interpretability of these networks. Additionally, the fusion of CNNs with other advanced technologies, such as reinforcement learning and natural language processing, opens up new frontiers for innovation.
3. Recurrent Neural Networks (RNNs) and Sequence Processing
Deep Neural Networks, specifically Recurrent Neural Networks (RNNs), have redefined sequence data analysis. RNNs excel in processing data with temporal dependencies, making them indispensable in natural language processing (NLP). They’ve significantly advanced speech recognition, machine translation, and sentiment analysis by adeptly capturing context and textual sequences.
Sequence data often involves information where the order and context of elements matter significantly. Consider natural language, where the meaning of a sentence can change entirely by reordering its words or phrases.
4. Deep Neural Networks and Long Short-Term Memory
Long Short-Term Memory Networks (LSTMs), a specialized variant of Deep Neural Networks, have emerged as a game-changing innovation in the realm of handling sequential data. These networks have successfully addressed the vanishing gradient problem, a critical challenge in training deep networks, and possess the unique capability to capture long-range dependencies within data sequences.
LSTMs have demonstrated their versatility by finding applications in diverse domains, ranging from finance for predicting stock prices and healthcare for patient monitoring to autonomous driving for accurately forecasting traffic patterns.
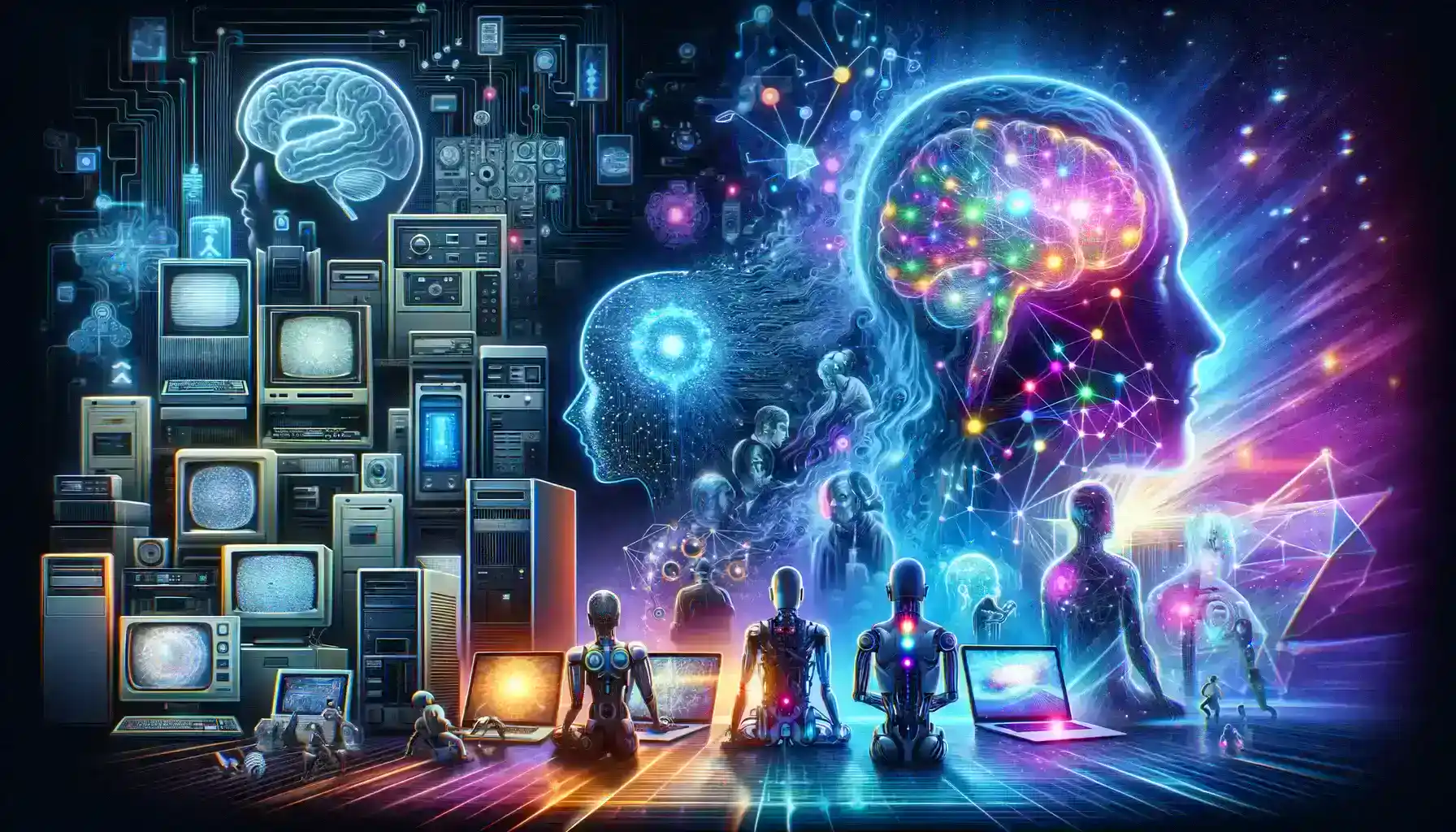
5. Generative Adversarial Networks (GANs)
Deep Neural Networks, particularly in the context of Generative Adversarial Networks (GANs), have sparked a revolution in data generation and manipulation. GANs consist of two neural networks, a generator, and a discriminator, engaged in a competitive process.
Deep Neural Networks within GANs are responsible for generating realistic data, including images, audio, and text, and have played a transformative role in fields such as image synthesis, style transfer, and even art generation.
6. Transfer Learning and Pre-trained Models
Transfer learning has emerged as a groundbreaking technique harnessing the capabilities of pre-trained DNNs, driving substantial improvements in the efficiency and effectiveness of deep learning models. Exemplified by models like BERT and GPT-3, which have been meticulously pre-trained on extensive datasets, this approach empowers AI systems with the unique capability to seamlessly transfer knowledge and contextual understanding to novel tasks.
The result is an unprecedented level of adaptability and accessibility, rendering these models invaluable across a diverse spectrum of applications, ranging from intricate language understanding tasks to nuanced sentiment analysis.
7. Reinforcement Learning and the Path to AGI
Deep Neural Networks are pivotal in the realm of reinforcement learning, playing a fundamental role in the development of Deep Q-networks (DQNs). These DQNs have showcased exceptional capabilities in mastering intricate tasks, marking a significant stride toward realizing the vision of Artificial General Intelligence (AGI).
The application of Deep Neural Networks in reinforcement learning becomes evident through achievements like AlphaGo, where these networks have not only matched but exceeded human performance in complex board games.
Ethical and Social Considerations
The widespread adoption of Deep Neural Networks has given rise to profound ethical and social considerations. These considerations encompass concerns related to potential biases present in training data, privacy implications, and the responsible lifecycle of AI systems.
Central to the discourse surrounding DNNs is the imperative to establish and maintain fairness, transparency, and accountability in their development and utilization.
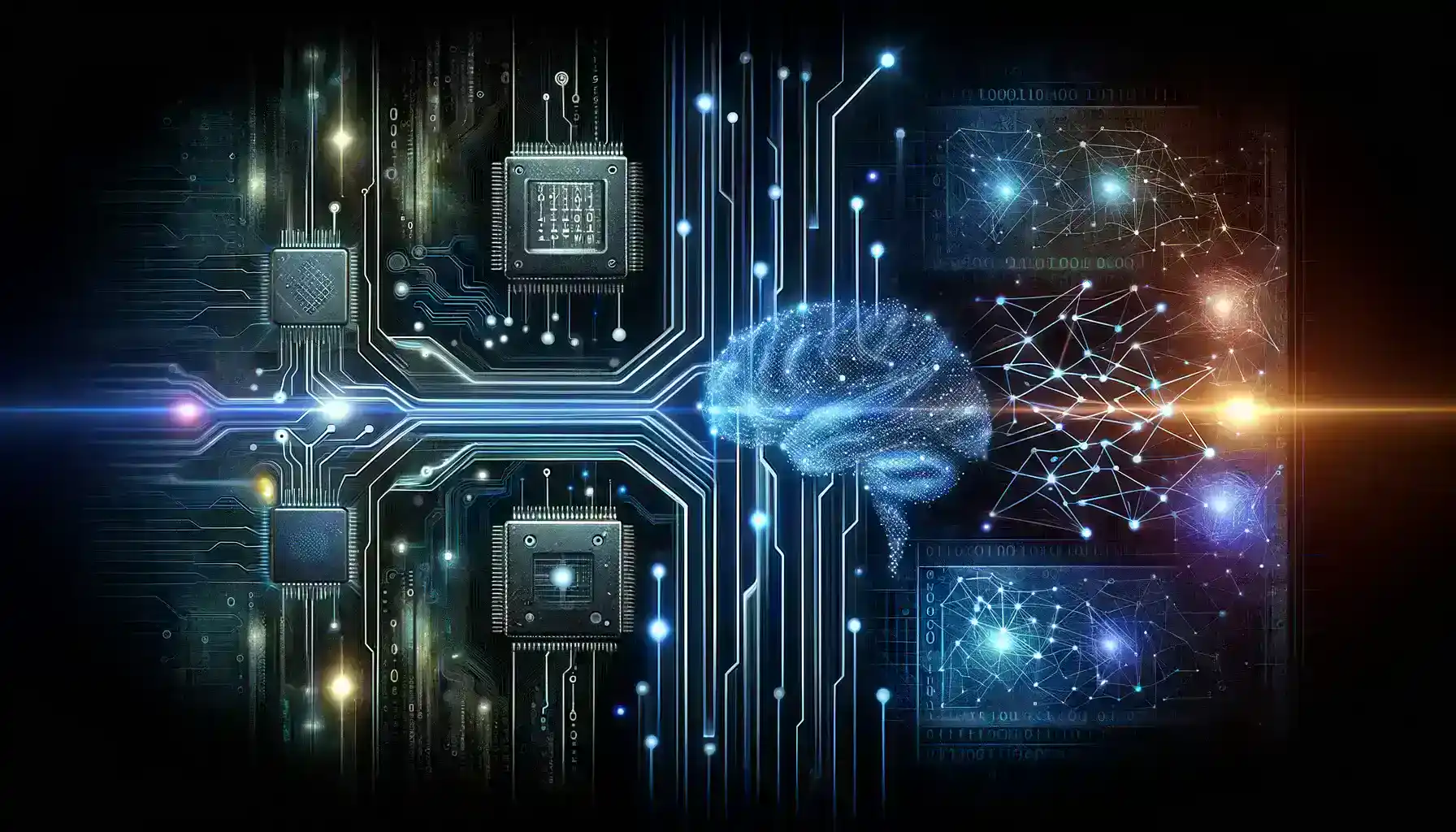
Future Prospects and Limitless Possibilities
The prospects and limitless possibilities presented by DNNs are nothing short of transformative. Here, we delve into the profound impact and the exciting opportunities that lie ahead:
- Energy Optimization: Deep Neural Networks are playing a vital role in optimizing energy consumption and sustainability efforts. They help in modeling and forecasting energy usage patterns, enabling smarter grid management and reducing energy wastage. DNNs are also applied in designing energy-efficient buildings and systems, contributing to a more sustainable future.
- Scientific Discoveries and Research: In the realm of scientific research, DNNs are assisting scientists in processing and analyzing vast datasets. Whether it’s in genomics, astronomy, or climate modeling, Deep Neural Networks aid researchers in pattern recognition, data analysis, and hypothesis generation. They accelerate the pace of discovery and innovation across various scientific disciplines.
- Natural Language Processing and Human-Machine Interaction: Natural language understanding and generation, powered by DNNs, are enhancing human-machine interaction. We can expect more advanced virtual assistants, chatbots, and language translation systems that facilitate seamless communication between humans and machines. This has implications for customer service, education, and cross-cultural collaboration.
- Robotics and Industrial Automation: DNNs are at the forefront of robotics and industrial automation. They enable robots to perform complex tasks with precision and adapt to dynamic environments. In manufacturing, logistics, and agriculture, Deep Neural Networks enhance efficiency, safety, and productivity.
- Entertainment and Creative Industries: DNNs are not limited to practical applications; they are also driving creativity and entertainment. They can generate art, music, and even storytelling. DNNs are being used to create immersive virtual worlds, enhance video games, and personalize content recommendations, offering new dimensions to the entertainment industry.
DNNs continue to be at the forefront of deep learning’s evolution, driving innovation and expanding the horizons of AI. The fusion of DNNs with other cutting-edge technologies like quantum computing and neuromorphic hardware holds immense promise for the future. The potential applications are boundless, from healthcare to autonomous systems, and scientific discovery to creative expression.
Deep Neural Networks are the keystones upon which the edifice of deep learning stands. As we embark on this journey of exploration and innovation, we are reminded of the transformative power they hold and the endless possibilities they offer for reshaping our world.
Conclusion
The journey through the evolution of deep learning and its game-changing breakthroughs has illuminated the extraordinary impact of this field on the landscape of artificial intelligence. From the birth of Deep Neural Networks to the rise of specialized architectures like Convolutional Neural Networks (CNNs) and Recurrent Neural Networks (RNNs), from the creative power of Generative Adversarial Networks (GANs) to the potential of reinforcement learning for achieving Artificial General Intelligence (AGI), deep learning has left an indelible mark on technology and society.
Yet, it is important to recognize that with great power comes great responsibility. As we navigate the limitless possibilities opened up by deep learning, we must also grapple with ethical considerations, privacy concerns, and the responsible development and deployment of AI technologies. The future of deep learning lies in our ability to harness its potential for the betterment of humanity, while also ensuring that it serves as a force for good.
In this ever-evolving landscape, the journey of deep learning is far from over. It is a journey marked by continuous innovation, collaboration, and the relentless pursuit of understanding and replicating human intelligence. As we stand at the threshold of an exciting future, we are reminded that the true power of deep learning lies not just in the technology itself, but in our collective wisdom and responsibility to wield it wisely for the benefit of all.