Table of Contents
AI (Artificial Intelligence) architecture plays a pivotal role in the development and functionality of AI systems. It serves as the blueprint, the very foundation upon which intelligent machines and applications are constructed. Just as the architectural design of a building determines its stability, aesthetics, and functionality.
AI has witnessed an unprecedented surge in its adoption and application across various industries. This phenomenon is not coincidental but a direct result of advancements in AI architecture. AI has witnessed an unprecedented surge in its adoption and application across various industries. This phenomenon is not coincidental but a direct result of advancements in AI architecture.
1. Understanding AI Architecture
AI architecture, in the context of artificial intelligence, refers to the structural framework or blueprint upon which Artificial Intelligence systems and algorithms are built. It encompasses the fundamental design choices, components, and strategies that govern how AI models process information, make decisions, and perform tasks.
The Future of AI architecture determines the overall efficiency, scalability, and adaptability of Artificial Intelligence systems. It influences the model’s ability to learn, generalize, and make accurate predictions.
A well-designed AI architecture can significantly enhance the performance and capabilities of AI applications, making it a critical consideration for researchers, developers, and organizations seeking to harness the power of artificial intelligence.
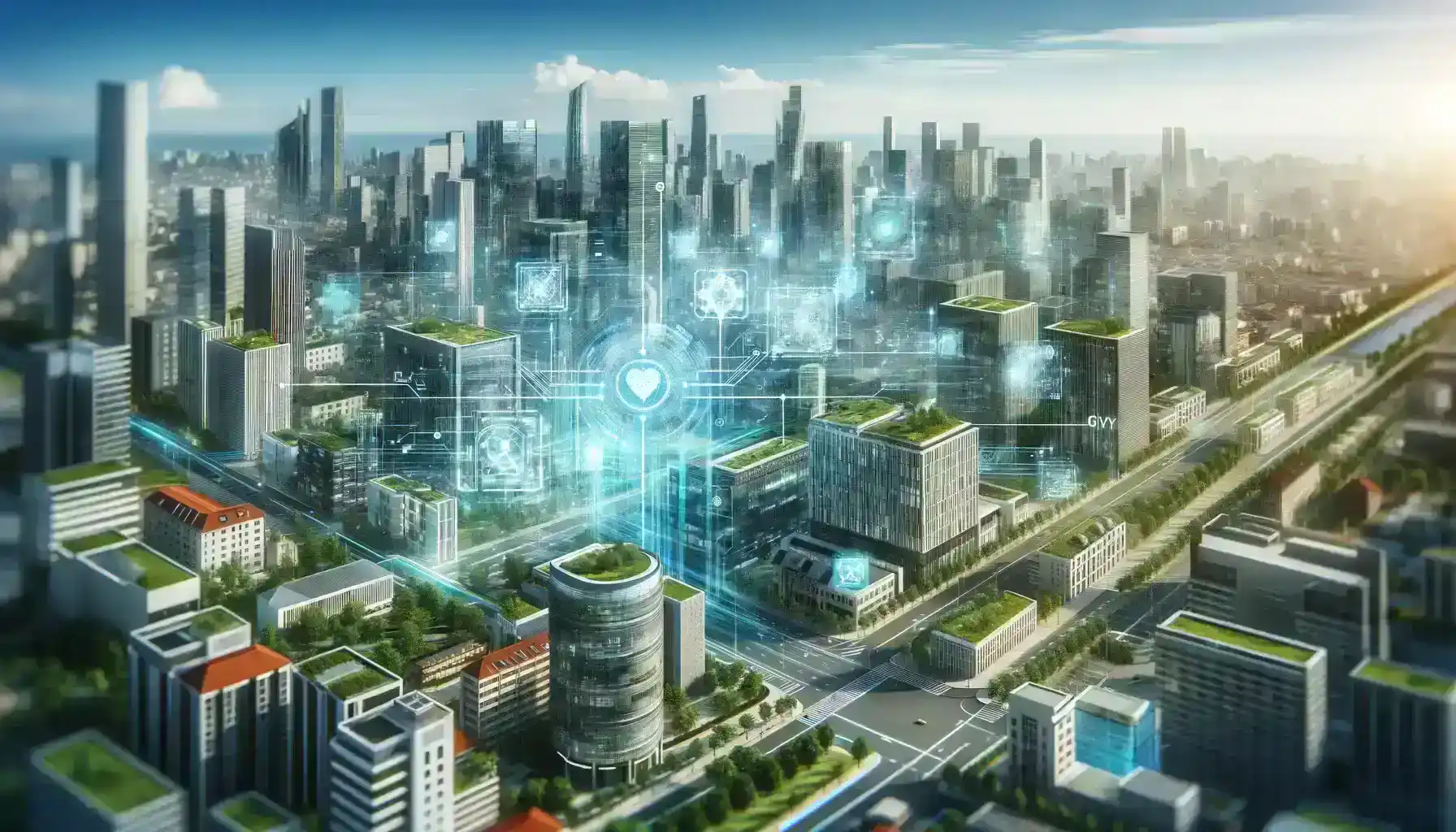
Principles of AI architecture
- This model comprises several essential components and principles that collectively shape the behavior and functionality of AI systems. These components include data input mechanisms, layers of neural networks, activation functions, loss functions, and optimization algorithms.
- Each component plays a distinct role in the AI model’s ability to process data, extract meaningful patterns, and generate insights.
- Principles such as supervised learning, unsupervised learning, and reinforcement learning underpin the learning processes within AI architecture.
- These principles guide how AI systems acquire knowledge from data and adapt their behavior over time.
- Additionally, architectural decisions regarding model depth, width, and connectivity impact the complexity and computational requirements of AI models.
2. Current State of AI Architechture
The field of AI architecture is marked by many frameworks and models that cater to various machine learning and deep learning tasks. Prominent among these are TensorFlow, PyTorch, and Keras, which serve as the foundational frameworks for developing and deploying AI models. These frameworks provide developers with essential tools and libraries to design, train, and evaluate the future of AI architectures effectively.
Strengths and Limitations of Current AI Architecture Approaches:
While current AI architecture frameworks and models have ushered in remarkable advancements, they come with their own set of strengths and limitations.
Strengths:
- Flexibility: Leading frameworks offer flexibility in model design, enabling developers to tailor architectures to their specific needs.
- Open-source community: The active open-source communities surrounding these frameworks foster collaboration, knowledge sharing, and rapid development.
- State-of-the-art performance: Models like Transformers have achieved state-of-the-art results in multiple benchmarks, pushing the boundaries of AI capabilities.
- Pretrained models: The availability of pre-trained models, such as BERT and GPT, simplifies the transfer learning process, reducing the training time for custom tasks.
Limitations:
- Computational resources: Many advanced AI model architectures demand substantial computational power, limiting accessibility for smaller research teams.
- Data requirements: Training deeply about the future of AI architectures often necessitates vast datasets, posing challenges in data collection and curation.
- Bias and fairness: AI models, including their architectures, may inherit biases from training data, raising ethical concerns that must be addressed.
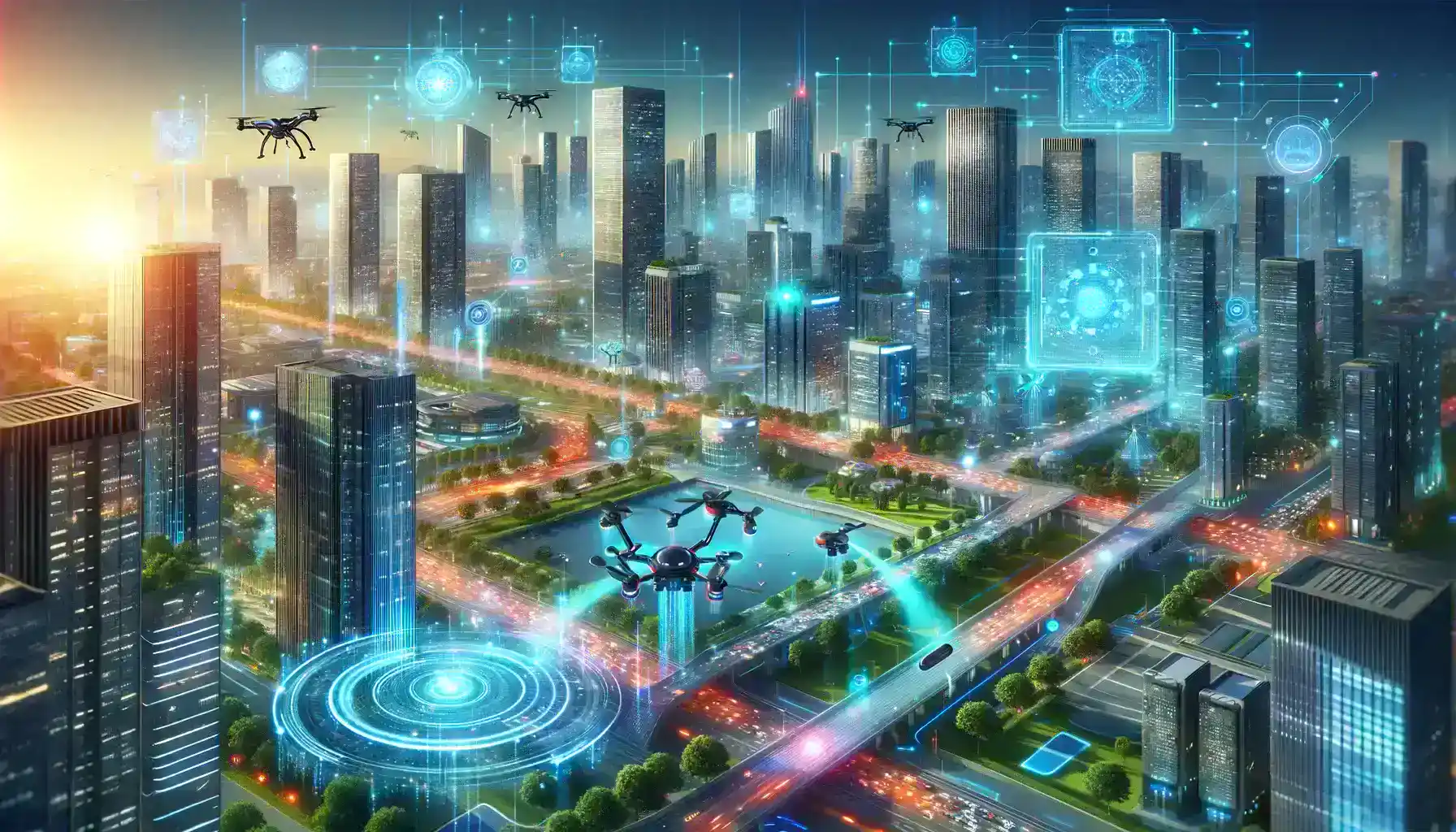
3. Emerging Trends
In this section, we will explore the dynamic landscape in the Future of AI architecture and explore the latest trends that are shaping the future of artificial intelligence.
- Federated Learning:
Federated learning is a transformative approach to the future of AI architecture that emphasizes privacy and decentralized model training. For instance, Google’s Federated Learning of Cohorts (FLoC) is revolutionizing online advertising by allowing browsers to personalize ads without exposing individual user data.
- Neural Architecture Search (NAS):
Neural Architecture Search is another burgeoning trend in AI architectures. NAS automates the process of designing and optimizing neural network architectures. It uses algorithms and strategies to discover high-performing neural network designs, saving significant time and resources.
- Explainable AI (XAI):
Explainable AI is gaining prominence as a crucial aspect in the future of AI architecture. This trend focuses on making AI systems more transparent and interpretable, allowing users to understand how decisions are made. XAI is particularly important in critical applications like healthcare and finance—companies like IBM with its AI OpenScale and Seldon with Alibi.
- Hybrid Cloud Architectures:
Hybrid cloud architectures combine on-premises data centers with cloud-based resources to optimize AI workloads. This approach provides flexibility, scalability, and cost-effectiveness. Companies like Amazon Web Services (AWS) and Microsoft Azure are offering hybrid solutions to the future of AI architecture requirements.
4. Predictions for the Future of AI Architecture
The future of AI architecture promises significant evolution. As computing power continues to advance, we can anticipate more complex and sophisticated AI model designs. The shift may involve the development of architectures that are more adaptive, dynamic, and capable of handling diverse data types.
Potential Breakthroughs in AI Architecture Design
Breakthroughs in AI model design are on the horizon. One of the most anticipated developments is the creation of more energy-efficient and sustainable architectures. Researchers are actively working on reducing the carbon footprint of AI systems.
Impact on Various Industries
- The advancements in AI model architectures will have a profound impact across a wide range of industries. Healthcare, for instance, will benefit from architectures that can analyze medical data more effectively, leading to more accurate diagnoses and personalized treatment plans.
- In the automotive sector, AI architectures will play a vital role in the development of autonomous vehicles, enhancing safety and efficiency.
- In finance, AI architecture will continue to revolutionize fraud detection, risk assessment, and algorithmic trading.
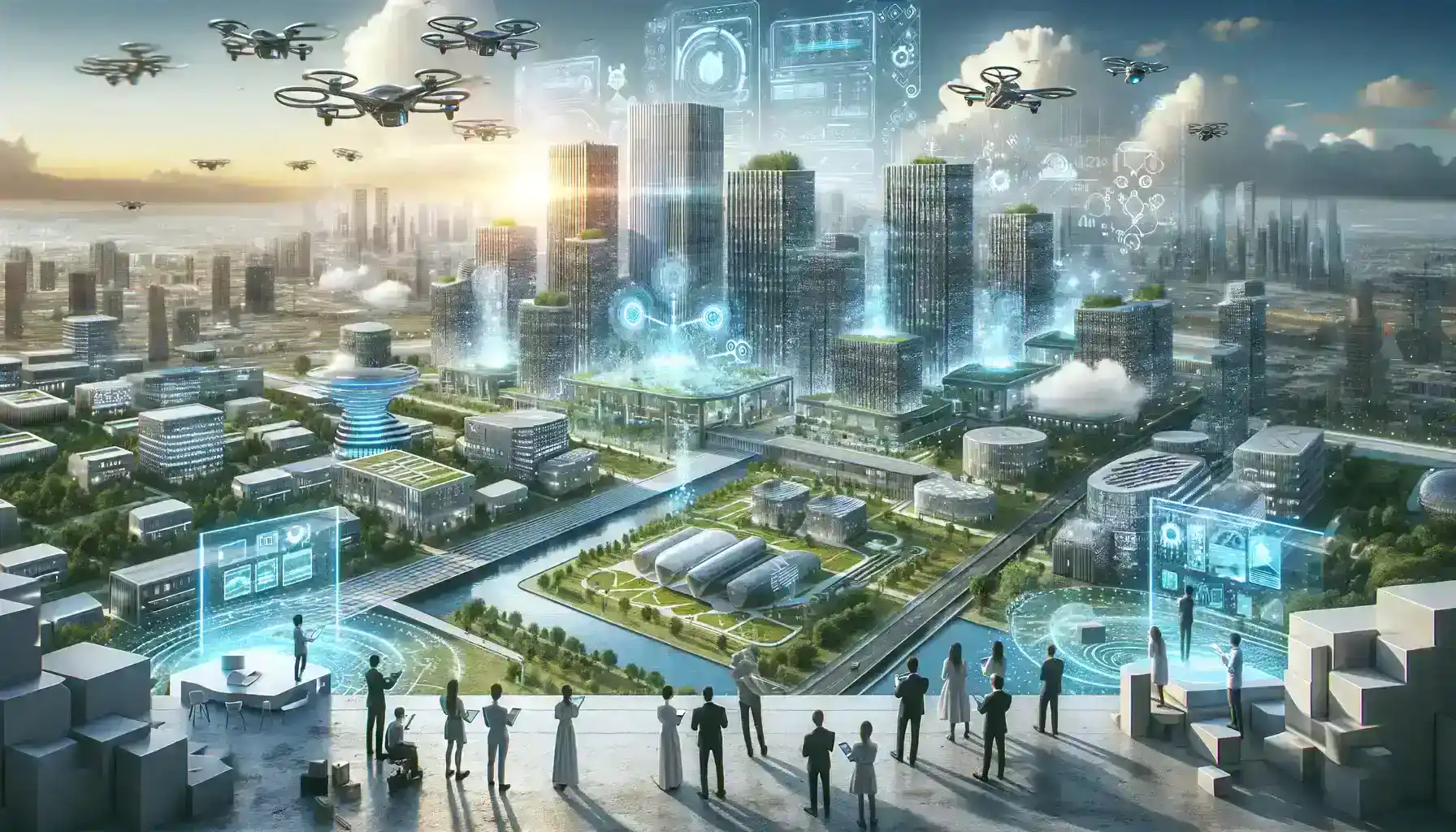
5. Challenges and Ethical Considerations
In this section, we will explore the multifaceted challenges and ethical considerations surrounding the development in the future of AI architectures. These aspects are crucial for building responsible and effective AI systems.
- Scalability: As AI applications become more complex, scaling AI model architectures to handle larger datasets and increased computational requirements becomes a significant challenge.
- Resource Constraints: Balancing the need for powerful hardware with the practical limitations of available resources is an ongoing challenge in AI architecture design.
- Interoperability: Ensuring that different AI systems and architectures can seamlessly work together and exchange information is essential for the future of AI.
- Security: Protecting AI architectures from malicious attacks and vulnerabilities is paramount, especially as AI becomes more integrated into critical systems.
Ethical Considerations
The ethical considerations will go hand in hand with the challenges.
- Bias and Fairness: AI systems can inadvertently perpetuate biases present in training data, leading to unfair and discriminatory outcomes. Addressing bias and ensuring fairness in AI model architectures is a critical ethical concern.
- Transparency and Explainability: As AI systems become more complex, they can be perceived as “black boxes” where it’s challenging to understand how decisions are made. Ensuring transparency and explainability in AI architecture is crucial for trust and accountability.
- Privacy: AI systems often require access to sensitive data. The future of AI architectures should prioritize robust privacy measures to protect user data.
- Accountability: Determining responsibility when AI systems make decisions is a growing ethical challenge. Who is accountable for the actions of AI? This is a question that needs to be addressed.
Overcoming Challenges and Ethical Considerations
To tackle these challenges and uphold ethical standards in development, several strategies can be employed:
- Research and Innovation: Ongoing research and innovation in the future of AI architectures are essential to finding solutions to scalability, resource constraints, and interoperability challenges.
- Algorithmic Fairness: Implementing fairness-aware algorithms and conducting thorough bias audits during model training can mitigate bias and fairness issues.
- Interdisciplinary Collaboration: Collaboration between AI experts, ethicists, and policymakers can help in creating guidelines and regulations to ensure the future of AI architectures.
- Ethics by Design: Incorporating ethical considerations into the design process of architecture from the outset can prevent many ethical issues from arising in the first place.
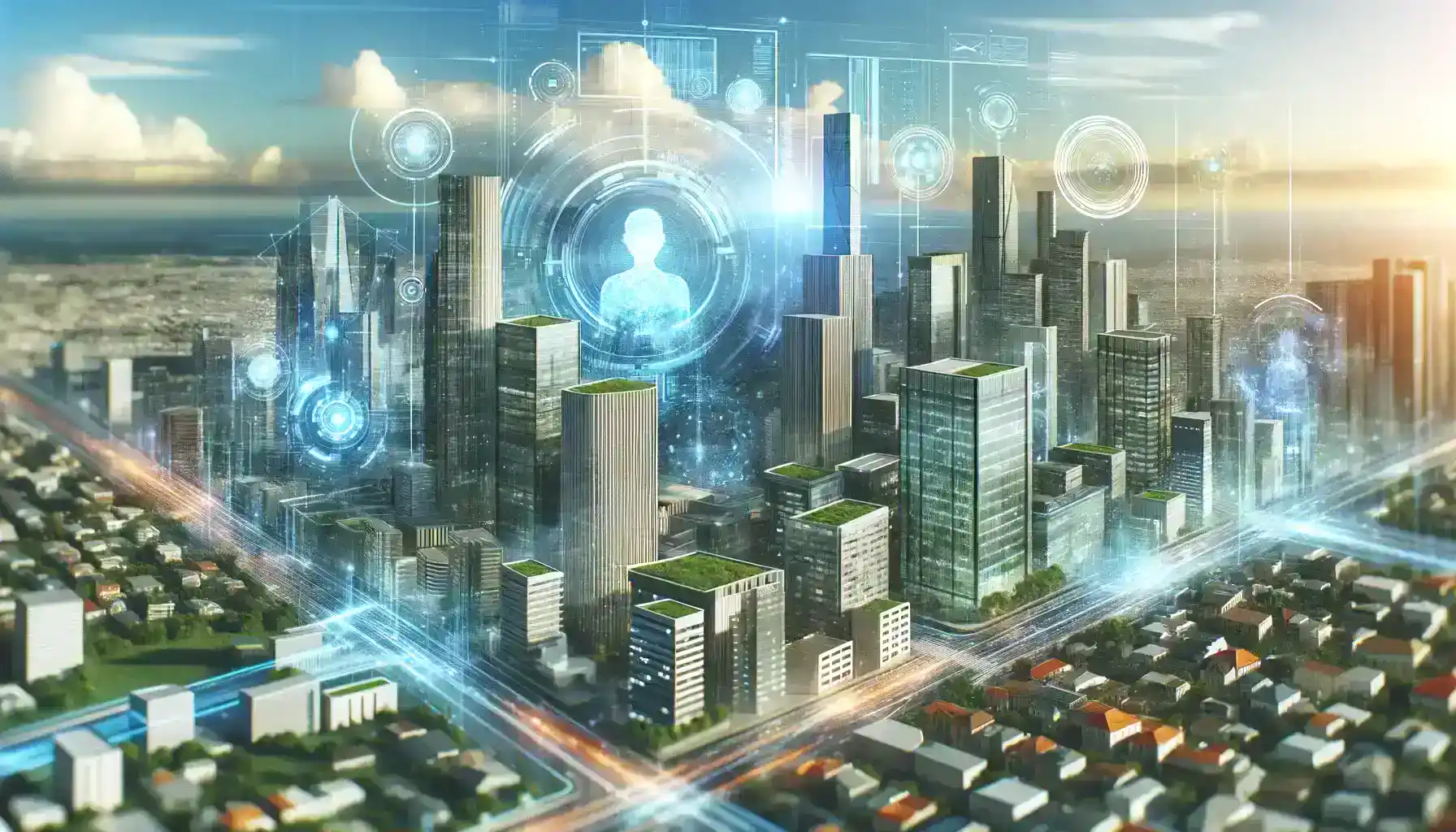
Conclusion
The future of AI architecture, we’ve delved into the fundamental role that AI architecture plays in shaping the trajectory of artificial intelligence. It is evident that as AI continues to permeate every facet of our lives, the evolution of its architecture is both imminent and transformative.
It is on the cusp of remarkable transformations, from architectures that adapt dynamically to the data they encounter to more sustainable and energy-efficient designs. Breakthroughs in neural network architectures will empower AI systems to achieve feats that were once thought impossible.
The impact of these architectural advancements will be felt across diverse industries. Healthcare will experience more accurate diagnoses and personalized treatments. Autonomous vehicles will become safer and more efficient. Every sector, from manufacturing to agriculture, will harness the power of AI architecture to optimize processes and drive innovation.
In this ever-evolving landscape, staying informed and adaptive is key. The Journey of architecture embarks, it is filled with endless possibilities and opportunities for positive change. The road ahead is exciting, and the future of AI architecture is bright.