Table of Contents
The rapid proliferation of artificial intelligence (AI) technologies has been nothing short of remarkable. From self-driving cars to advanced healthcare diagnostics, AI has demonstrated its potential to revolutionize various industries and improve our daily lives. The term “Green AI” has emerged as a response to the environmental challenges posed by the ever-increasing energy appetite of AI systems.
Green AI
Green AI can be defined as an approach to developing, deploying, and utilizing artificial intelligence in a manner that prioritizes sustainability and minimizes energy consumption and carbon emissions. It is a multidisciplinary effort that combines elements of hardware design, software development, data management, and renewable energy integration to make AI systems more eco-friendly.
Core Principles of Green AI
- Energy Efficiency: One of the fundamental principles of Greening AI is optimizing energy usage. This involves developing energy-efficient hardware components, such as GPUs and TPUs, and implementing energy-saving algorithms to reduce the power requirements of AI systems.
- Sustainable Hardware: Greening AI emphasizes the use of sustainable and eco-friendly materials in the manufacturing of AI hardware. This includes the reduction of hazardous materials, the promotion of recycling, and the development of hardware that can be easily upgraded and maintained.
Reducing the Carbon Footprint of AI
- Green AI seeks to achieve a significant reduction in the carbon footprint of artificial intelligence through a combination of the principles mentioned above. By optimizing hardware, algorithms, and data management, AI systems can operate more efficiently, consuming less power for the same tasks.
- Green AI envisions a future where AI technologies not only advance rapidly but also do so in harmony with the environment. It is about creating a sustainable AI ecosystem that aligns technological progress with the goals of environmental preservation and energy efficiency.
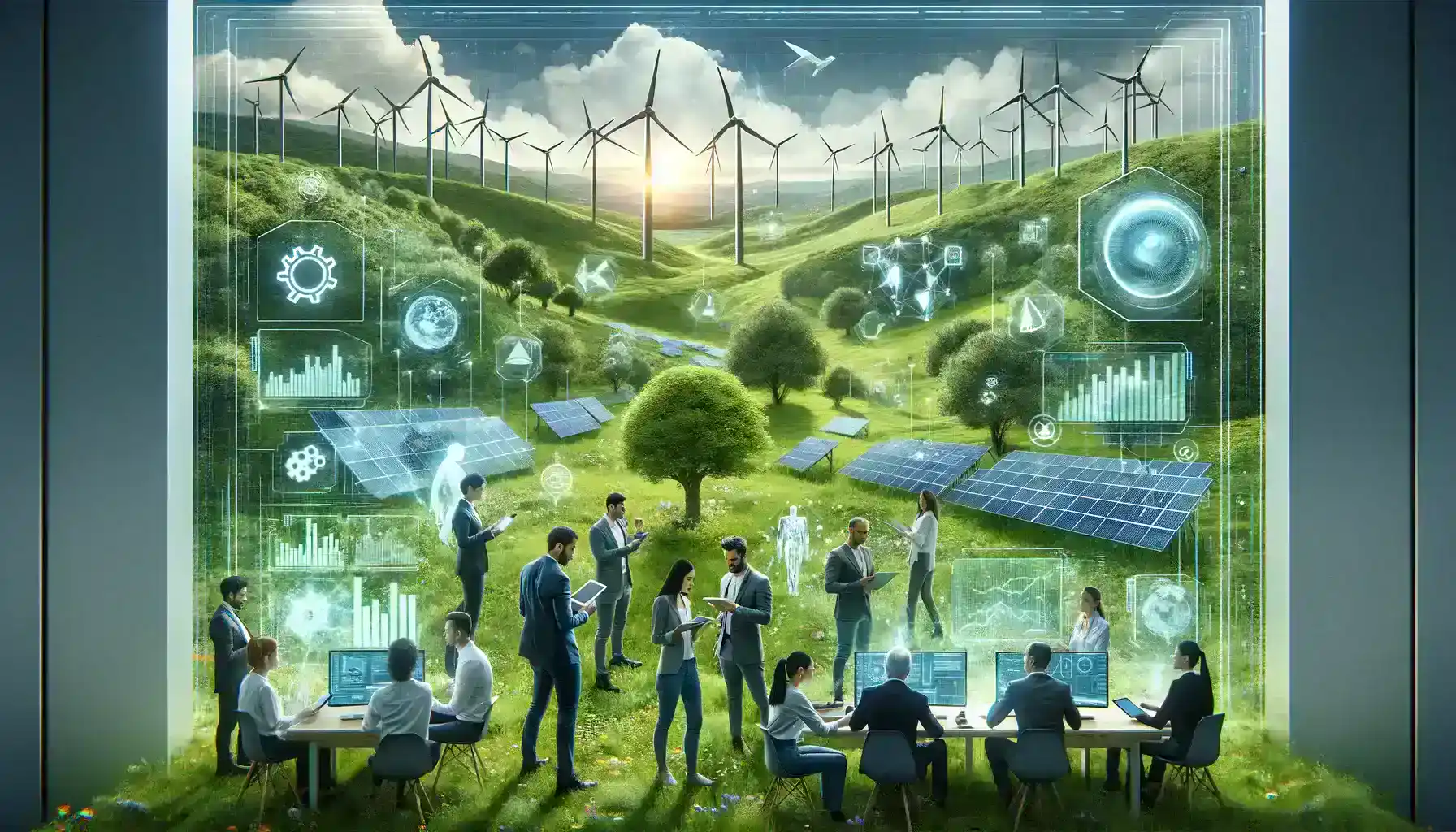
Strategies for Energy Mitigation
In the “Strategies for Energy Mitigation” section, we will explore the various approaches and techniques that can be employed to make AI systems more energy-efficient.
1. Hardware Optimization: Hardware optimization plays a pivotal role in improving the energy efficiency of AI systems. This involves designing and selecting hardware components that consume less power while maintaining or enhancing performance. Some key hardware optimization strategies include:
- Energy-efficient Processors: Using energy-efficient central processing units (CPUs) and graphics processing units (GPUs) can significantly reduce power consumption. Specialized AI hardware, such as TPUs (Tensor Processing Units) and FPGAs (Field-Programmable Gate Arrays), are also designed for AI workloads with an emphasis on energy efficiency.
- Cooling Solutions: Efficient cooling mechanisms, like liquid cooling or advanced cooling fans, can help maintain optimal operating temperatures and prevent excessive power consumption due to overheating.
2. Algorithmic Improvements: Optimizing the algorithms used in AI models is another critical aspect of reducing energy consumption. Here’s how algorithmic improvements contribute to energy mitigation:
- Sparse Models: Sparse models are designed to use fewer parameters, which reduces computational requirements and power consumption during training and inference. Techniques like model pruning and quantization help create more energy-efficient AI models.
- Efficient Architectures: Researchers are continually developing more efficient neural network architectures, like MobileNet and EfficientNet, specifically designed to deliver high accuracy with lower computational demands.
3. Data Management: Effective data management is essential for energy-efficient AI systems. Data-related tasks, such as storage, retrieval, and preprocessing, can be resource-intensive. Here’s how data management contributes to energy mitigation:
- Data Compression: Compressing datasets and model weights can reduce the amount of data that needs to be transferred and stored, resulting in lower energy consumption. Techniques like quantization and Huffman coding are commonly used for this purpose.
- Data Pipelines: Implementing efficient data pipelines that preprocess and feed data to AI models in a streamlined manner can minimize resource wastage and energy usage.
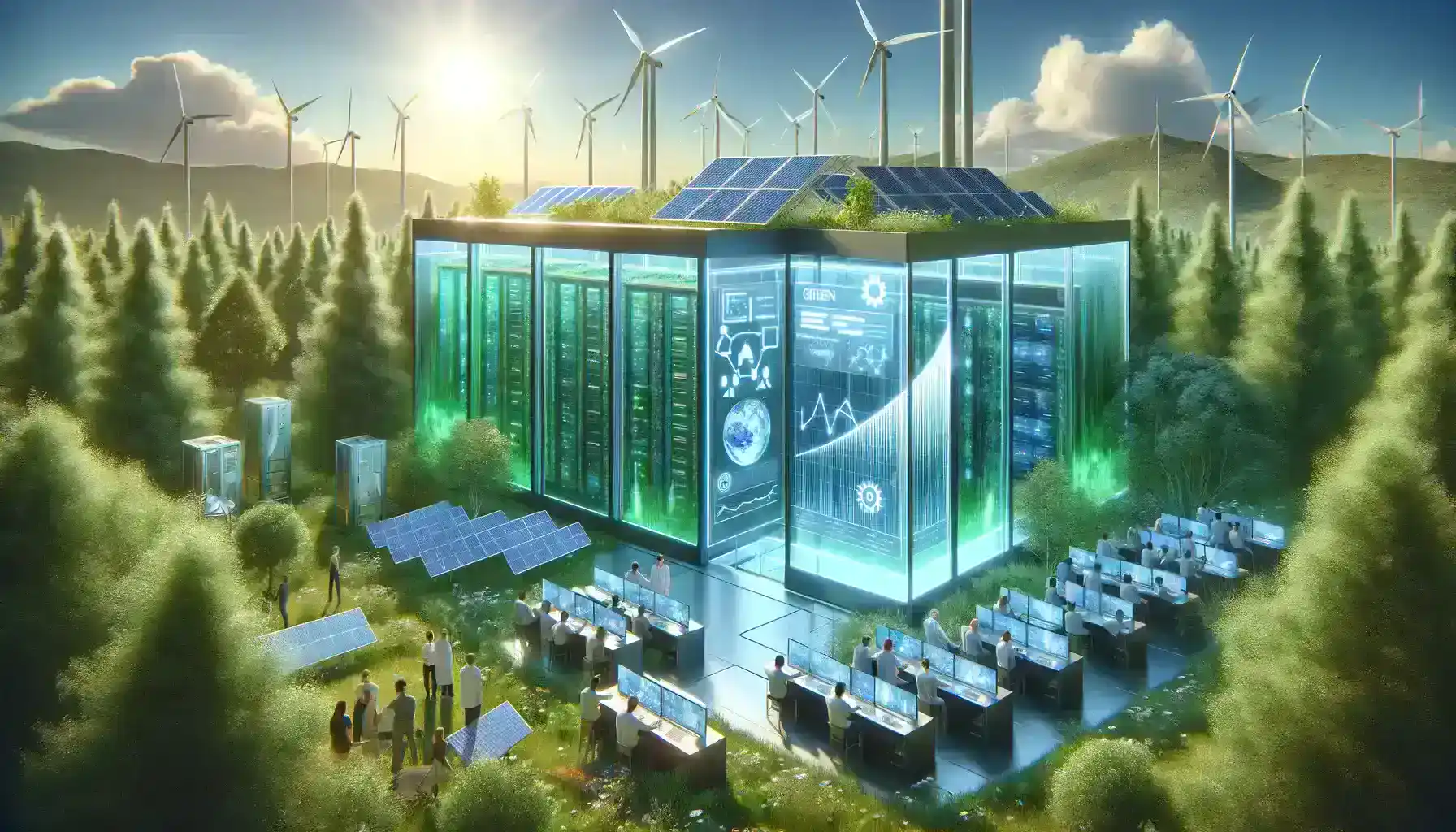
Hardware Innovations
In recent years, one of the significant strides in mitigating the energy consumption of AI has been the development of energy-efficient hardware components.
Advancements in Energy-Efficient Hardware Components
- GPUs and TPUs: GPUs have long been a staple in AI and machine learning for their parallel processing capabilities. However, traditional GPUs were not optimized for energy efficiency. With the growing concern about energy consumption, GPU manufacturers have been working diligently to create more energy-efficient models. Similarly, TPUs, which are custom-built for machine learning tasks, are designed with a focus on energy efficiency, making them an attractive choice for AI applications.
- Reduced Power Consumption: Newer generations of GPUs and TPUs are engineered to provide better performance per watt. This means that they can deliver the same computational power as their predecessors while consuming significantly less energy.
- Google’s TPU Deployment: Google is a pioneer in the development of energy-efficient hardware for AI. They designed their TPUs specifically to accelerate machine learning workloads while minimizing power usage. Google’s deployment of TPUs in their data centers has resulted in remarkable energy savings. By replacing traditional GPUs with TPUs in their AI infrastructure, Google reduced the energy required for AI tasks, leading to a more environmentally friendly approach.
Renewable Energy Integration
In the quest to make artificial intelligence (AI) more environmentally friendly, one of the key strategies is the integration of renewable energy sources to power AI infrastructure. This approach holds immense importance as it aligns AI development with sustainability goals and helps mitigate the rising energy usage associated with AI technologies.
- Reducing Carbon Footprint: One of the primary benefits of renewable energy integration in AI is the significant reduction in carbon emissions. Traditional AI data centers are notorious for their energy consumption, often relying on fossil fuels.
- Cost Savings: Renewable energy sources have become increasingly cost-effective, making them a viable and economical choice for powering AI infrastructure. Organizations can not only reduce their environmental impact but also benefit from lower energy costs over time, thus achieving a dual advantage.
- Resilience and Reliability: Renewable energy sources are often more resilient to disruptions compared to centralized energy grids. This resilience ensures the uninterrupted operation of AI systems, even during power outages or grid failures, which is critical for applications where downtime can be costly or pose safety risks.
Challenges and Limitations
Green AI, while promising, faces several challenges and limitations that must be addressed for its widespread adoption.
- Complexity and Cost: Implementing energy-efficient AI solutions can be complex and costly, particularly for organizations with existing infrastructure. Upgrading hardware, optimizing software, and retraining models all require significant investments.
- Performance Trade-offs: Achieving energy efficiency may involve performance trade-offs. Some energy-saving algorithms or hardware optimizations might result in slightly reduced AI performance, which can be unacceptable in critical applications.
- Data Availability: Green AI relies on vast amounts of data for training and optimization. Access to clean and diverse datasets is crucial, but it’s often a challenge to obtain and maintain such data.
- Regulatory and Ethical Considerations: Regulations related to AI and environmental impact are evolving. Organizations must navigate the legal and ethical landscape surrounding AI, including data privacy and environmental regulations.
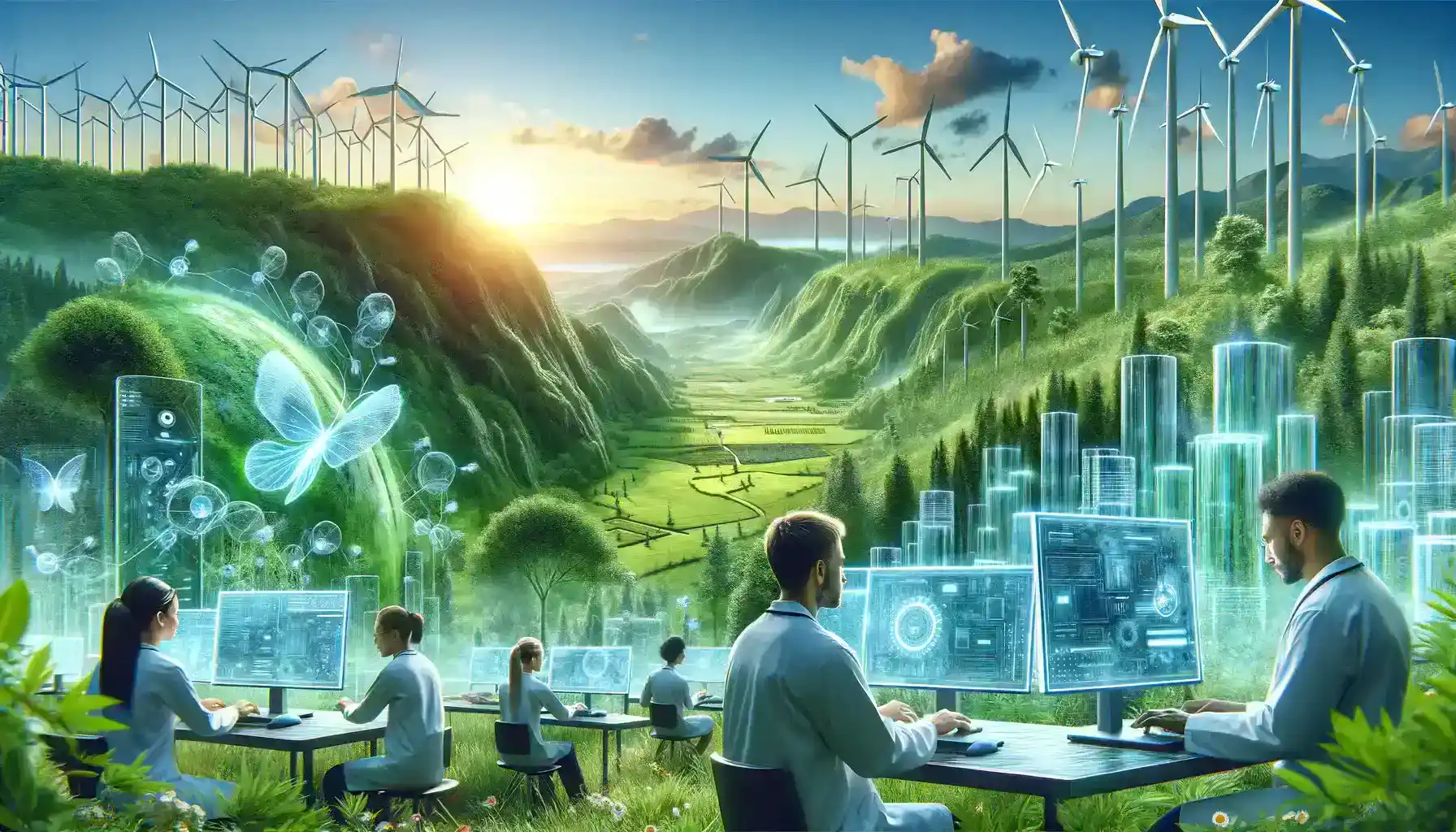
Future Outlook of Green AI and its Environmental Impact
Despite these challenges, Green AI holds great potential for reducing the environmental footprint of AI technologies.
- Improved Efficiency: Ongoing research and development will lead to more efficient AI algorithms and hardware. These advancements will help strike a balance between energy savings and performance.
- Wider Adoption: As the benefits of Green AI become more evident, more organizations will prioritize energy-efficient AI solutions. This will lead to a broader adoption of sustainable practices in AI development.
- Policy and Regulation: Governments and international bodies will likely establish clearer guidelines and regulations for AI’s environmental impact. This will encourage organizations to invest in Green AI technologies.
- Innovation in Renewable Energy: The integration of AI with renewable energy sources will continue to grow. Smart grids and energy management systems powered by AI will become more sophisticated, optimizing energy usage across various sectors.
Conclusion
In a world where artificial intelligence (AI) is becoming increasingly ubiquitous, the concept of “Green AI” and the strategies to mitigate rising energy usage within this field have taken on paramount importance. The journey towards making AI more environmentally friendly and energy-efficient is not just an option; it’s an imperative for a sustainable future.
From optimizing hardware and algorithms to the integration of renewable energy sources, the path to Green AI is multifaceted and promising. The adoption of renewable energy for AI offers economic advantages, with lower energy costs and the potential for long-term savings.
The significance of powering AI infrastructure with renewable energy sources cannot be overstated. By transitioning away from fossil fuels and embracing clean, renewable alternatives, we can substantially reduce AI’s carbon footprint.
As we conclude this exploration of Green AI, it’s evident that the fusion of artificial intelligence with sustainability is not only a technological necessity but also a moral imperative. The synergy between AI and renewable energy holds the promise of a more eco-conscious and efficient future.
Green AI isn’t just a strategy; it’s a transformative movement that seeks to harmonize the boundless potential of artificial intelligence with the needs of our planet. It’s a testament to our commitment to innovation, responsibility, and the preservation of the environment.