Table of Contents
What Is Narrow Artificial Intelligence?
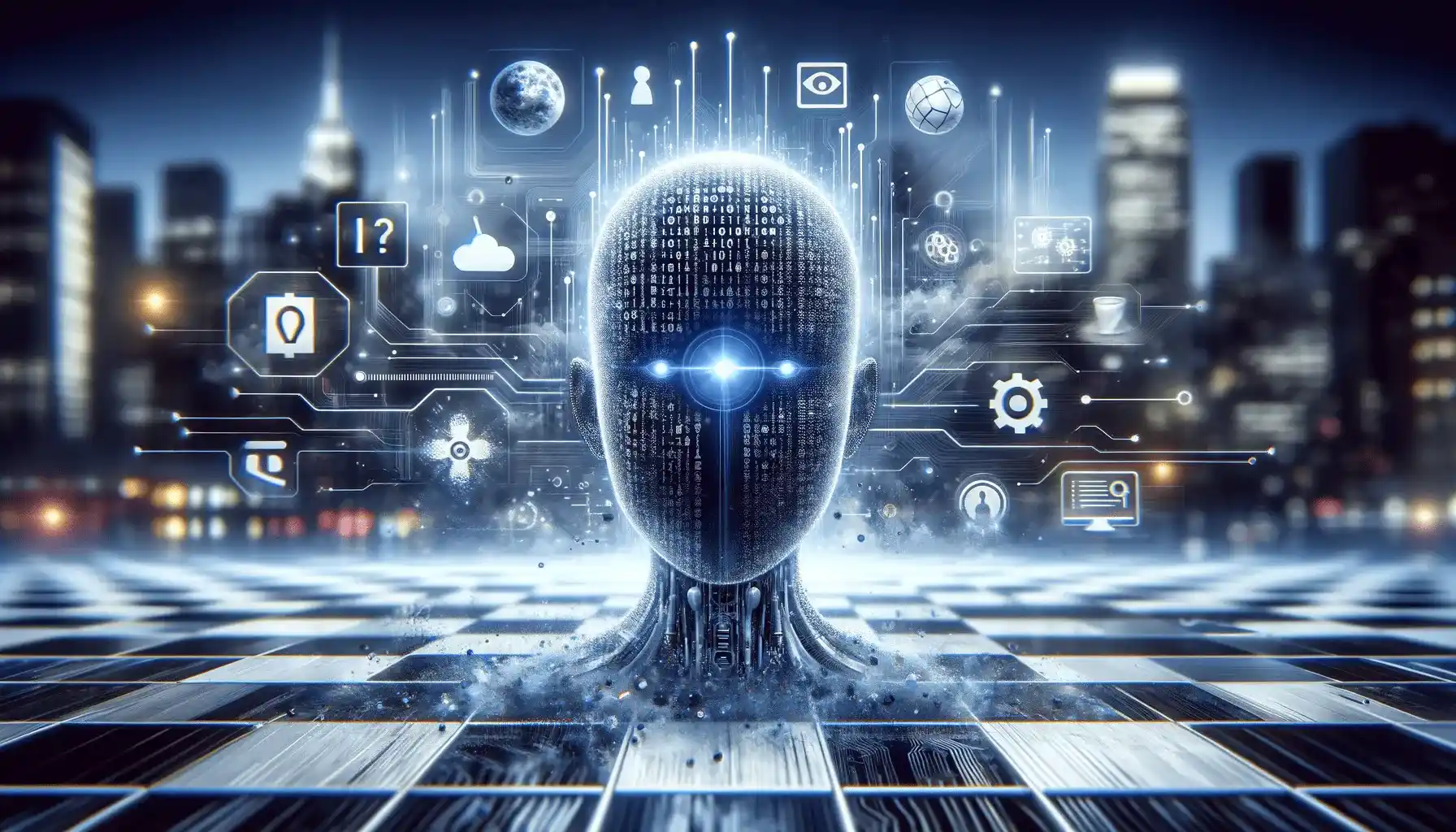
Artificial Narrow Intelligence (ANI), also known as Narrow AI, is specifically tailored to excel in performing single tasks, such as weather tracking, data analysis, or playing games like chess. Unlike General AI, ANI lacks self-awareness and the ability to exhibit genuine intelligence beyond its designated function. Criteria of ANI include virtual assistants like Siri and Google Assistant, which can understand and respond to human language within predefined parameters. However, they cannot think independently or understand abstract concepts. Even complex systems like self-driving cars operate on Narrow AI, relying on predefined algorithms to navigate their surroundings. While ANI systems can perform tasks efficiently within their defined contexts, they do not possess the cognitive flexibility of human intelligence.
Navigating Challenges in 2024
In 2024, Artificial Narrow Intelligence will face notable challenges. Data bias remains a key concern, where AI systems may produce unfair outcomes due to biases in training data. The lack of interpretability is another hurdle, as intricate AI models operate as “black boxes,” hindering transparency in decision-making processes. Ethical dilemmas, including privacy infringements and job displacement, persist as pressing issues in AI deployment. These challenges underscore the importance of addressing data bias, enhancing interpretability, and navigating ethical considerations to foster trust and maximize the potential of Narrow AI in various domains.
Virtual Assistants: Voice-activated assistants like Amazon’s Alexa and Apple’s Siri utilize Narrow AI to understand and respond to user commands, perform tasks, and provide information tailored to individual preferences.
Exploring the Benefits of Narrow AI
- Enhances Decision-Making Speed: Narrow AI expedites decision-making processes by swiftly processing data and executing tasks, surpassing human capabilities. For instance, IBM’s Watson aids doctors in making data-driven decisions promptly, revolutionizing healthcare efficiency and safety.
- Automates Mundane Tasks: Advancements in narrow Artificial Narrow Intelligence humans from tedious routines, simplifying daily life activities like online food ordering through virtual assistants. Technologies like self-driving cars liberate individuals from traffic stress, providing more leisure time.
- Catalyst for Advanced AI Development: Artificial Narrow Intelligence lays the groundwork for future AI iterations, such as general AI and super AI. Foundational technologies like speech recognition and computer vision, utilized by Google for video captioning, pave the way for more sophisticated AI applications.
- Superior Performance in Specific Tasks: Artificial Narrow Intelligence excels in specialized tasks, like cancer detection from medical images or predictive maintenance in manufacturing. These systems outperform humans in speed and accuracy, enhancing efficiency and productivity.
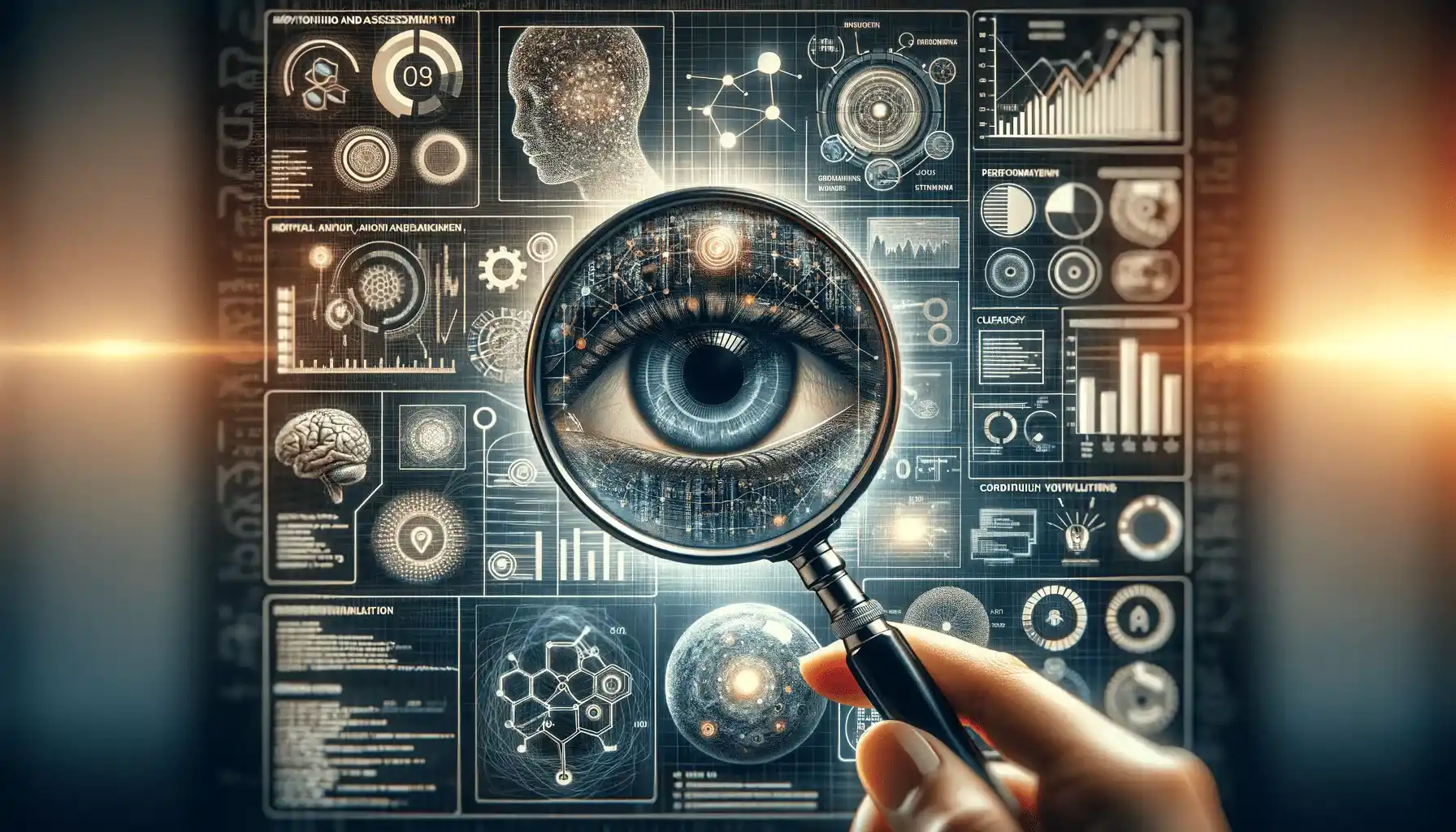
Best Practices for Harnessing the Power of Narrow AI
Data Quality Assurance: Data Quality Assurance involves meticulous verification and validation processes to ensure the integrity, diversity, and reliability of datasets used for AI training. By meticulously curating and cleansing datasets, organizations can mitigate bias and ensure that AI models accurately represent real-world scenarios. This practice enhances the accuracy and effectiveness of AI algorithms, leading to more reliable insights and predictions. Additionally, diverse datasets enable AI systems to generalize better and perform effectively across different contexts, contributing to improved decision-making and outcomes.
Explainability and Transparency: Explainability and Transparency in Narrow AI entail implementing mechanisms that allow users to understand AI decisions and receive clear explanations for them. This fosters trust and accountability by providing insight into how AI systems arrive at conclusions or recommendations. Transparent explanations enable users to assess the reliability and fairness of AI-driven outcomes, promoting confidence in the technology’s capabilities. By prioritizing explainability and transparency, organizations can enhance user trust, mitigate potential biases, and ensure responsible AI deployment.
Ethical guidelines: Ethical guidelines in AI development prioritize user privacy, fairness, and societal well-being. It involves ensuring that AI systems are designed and deployed in a manner that respects individual rights, avoids discrimination, and contributes positively to society. This includes transparent data handling practices, fair algorithms that do not perpetuate biases, and consideration of the broader societal impacts of AI applications. Adhering to ethical principles fosters trust among users, promotes responsible innovation, and mitigates potential harms associated with AI technologies.
Human-Centric Design: Human-centric design emphasizes understanding user behaviors, needs, and preferences to create AI solutions that enhance user experiences. By prioritizing a user-centric approach in AI development, organizations ensure that their solutions are intuitive, accessible, and tailored to meet the specific needs of users. This approach involves gathering user feedback, conducting usability testing, and iterating on designs based on user insights. Ultimately, human-centric design leads to more user-friendly and effective AI applications that drive greater satisfaction and adoption among users.
Monitoring and Assessment: Monitoring and Assessment involves the systematic use of metrics to evaluate the effectiveness of AI training and monitoring processes regularly. By implementing this practice, organizations can ensure ongoing performance optimization of their AI systems. Through the continuous assessment of key metrics such as accuracy, efficiency, and reliability, any deviations or anomalies can be promptly identified and addressed. This proactive approach enables organizations to maintain the quality and effectiveness of their AI deployments, ultimately maximizing the value derived from narrow AI technologies.
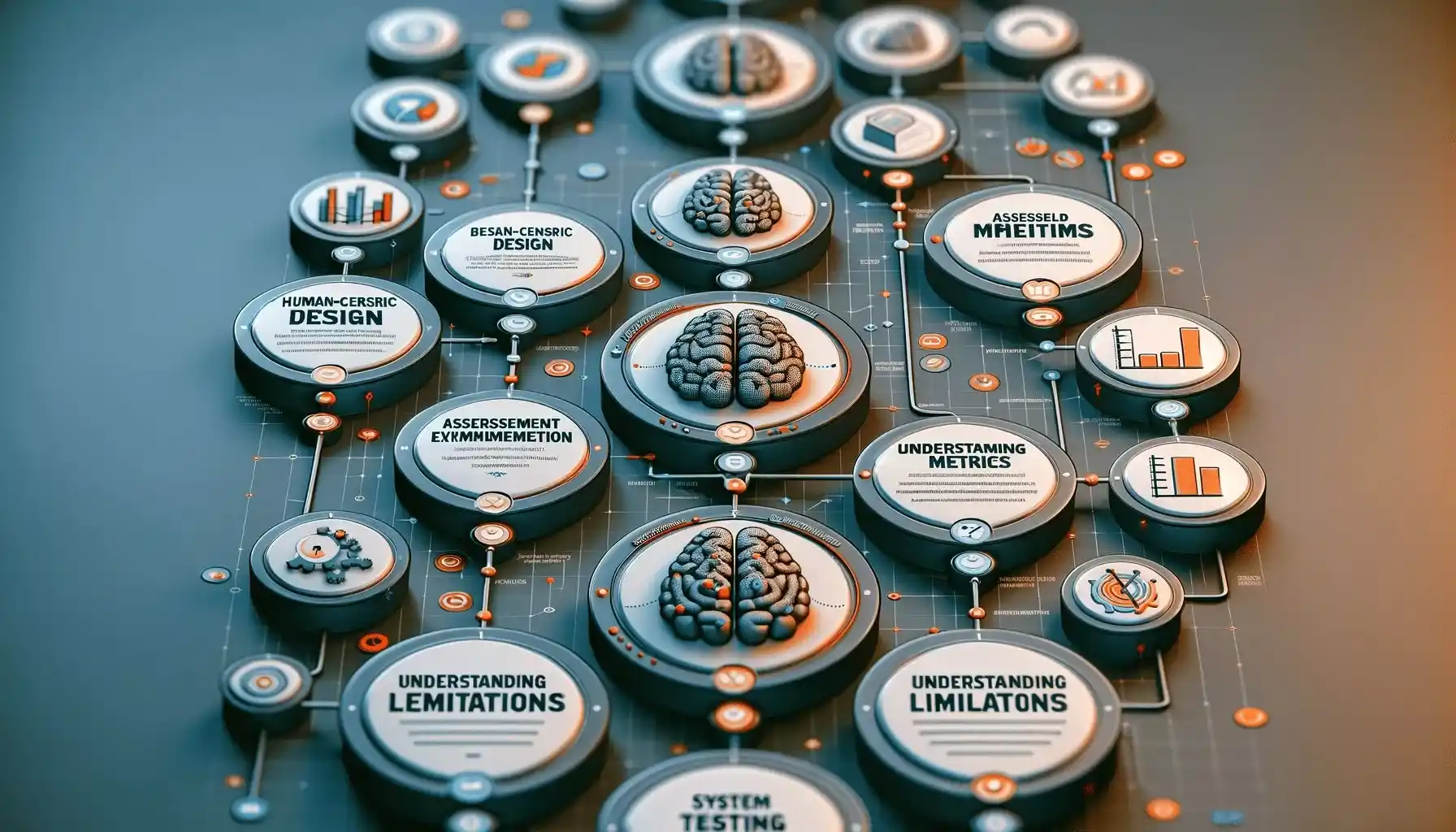
Continuous Evaluation: Continuous evaluation involves regularly examining raw data to identify any issues or limitations within AI datasets and models. By conducting periodic assessments, organizations can ensure that their AI systems remain effective and accurate over time. This process allows them to detect any biases, inaccuracies, or shortcomings in the data or model, enabling them to take corrective actions as needed. Ultimately, continuous evaluation helps maintain the reliability and performance of AI applications, ensuring that they continue to deliver valuable insights and outcomes for the organization.
Rigorous testing: It’s essential to ensure the functionality and reliability of AI systems. It involves conducting comprehensive assessments across diverse scenarios and conditions to verify that the system performs as intended. Through rigorous testing, potential flaws, errors, or inconsistencies can be identified and addressed, enhancing the overall quality and effectiveness of the AI solution. By thoroughly evaluating the system’s performance through testing, organizations can instill confidence in its capabilities and mitigate the risk of unexpected failures or inaccuracies in real-world deployments.
Conclusion
Artificial Narrow Intelligence (ANI) presents a promising avenue for innovation and advancement across various domains, offering unparalleled capabilities to expedite decision-making, automate mundane tasks, and drive superior performance in specialized areas. However, as we navigate the landscape of Narrow AI in 2024, it is imperative to address critical challenges such as data bias, interpretability limitations, and ethical considerations to foster trust and maximize its potential impact. By adhering to best practices such as ensuring data quality assurance, prioritizing explainability and transparency, and adhering to ethical guidelines, organizations can harness the power of Narrow AI responsibly and ethically.
Additionally, adopting a human-centric design approach, monitoring and assessing AI performance, and continuously evaluating raw data are essential strategies to maintain the reliability and effectiveness of AI systems over time. Through concerted efforts to overcome challenges and implement best practices, Narrow AI can truly revolutionize industries, drive innovation, and positively impact society in the years to come.