Table of Contents
Below are the 10 powerful ways predictive analytics transforms retail, with the fast-changing world of retail, businesses never stop to look for something new to be more competitive and meet the increasingly dynamic consumer expectations. This has proven to be a game-changer in that it will let one use data and be spot-on for future identification of trends-the future. It would also serve to ensure the empowerment of the retail organization through advanced algorithms and machine learning into data-driven and therefore better decisions for improvement in operations, customer connection, and increased profit levels.
Unlocking Predictive Analytics: What It Is and Why It Matters
Predictive Analytics is the process of making predictions based on historical data, statistical algorithms, and machine learning techniques. It enables businesses to determine patterns and trends hidden in enormous datasets and helps predict future customer behavior, sales trends, and operational needs. This technology provides retailers with the ability to remain ahead of market demands while ensuring good anticipation of consumer preferences. The information transforms raw data into actionable insights in myriad industries, particularly in the retail trade; thus, optimizing operations, personalizing services, and predicting market shifts.
The Power Behind Predictive Analytics: How It Works
Fundamentally, this technology collects substantial quantities of historical data from diverse sources, including customer purchase records, online activities, and external market trends, subsequently employing algorithms to recognize patterns. Subsequently, machine learning models evaluate these identified patterns against new datasets to anticipate future occurrences. Such predictions may encompass a spectrum from estimating sales volume to detecting potential customer attrition. The accuracy of these predictions improves constantly since the system learns from new inputs of data. By processing large data volumes, it empowers retailers to make data-driven decisions and gain a competitive edge.
Predictive Retail Analytics Explained
It is a mix of information from multiple channels, point-of-sale data, customer engagement, social network behavior, and website activities, it takes advanced machine algorithms to discern these patterns about what would happen in the future. Be it some demand prediction, customer churn rate, the percentage about retaining some of the current customers, or some suitable price, always putting the retail player at a step beyond the other competitor. It enables proactive decision-making by transforming data into actionable insights that help improve customer experience, increase sales, and enhance business efficiency.
How Predictive Models Drives Smart Decisions in Retail
For the retail industry this technology changes the orientation of decision-making by telling future trends through historical consumer data. It gives retailers real-time sight of optimum inventory management consumer preferences and price elasticity in consumption. Hence, this technology predicts demand and therefore assists retailers in developing efficient supply chains, which translates to reduced waste and improved shop experiences for consumers. The risk is, therefore, minimized while profit is maximized for any business entity with smart, proactive decisions on competitive position in a highly dynamic market.
Data-Driven Insights in Retail: Key Trends and Impact
Usage of Predictive Analytics in Retail: More than 80% of retailers have already started using predictive models to get insights from their data. This technology helps businesses anticipate customer behaviors, and trends, and optimize inventory management. Furthermore, 74 percent of retail decision-makers identified that handling returns on online sales is difficult. It can increase margins while lessening the strain from this difficulty by including predictive models in their operations. [businesswire]
Operational Costs Impact: Predictive technologies can bring about a reduction of up to 15% in operational costs since it helps a business optimize inventory management, reduce guesswork in terms of customer demands, and provide more optimal resource utilization. Additionally, it can allow a business to find loopholes and gaps that enhance its decision-making and better manage its resources. [quantzig]
ROI of Predictive Analytics: Any retailer using the solution of predictive models experiences a return on investment (ROI) of up to 300%.The ability to predict trends, personalize customer experiences, and optimize product offerings based on predictive analytics significantly boosts sales. [quantzig]
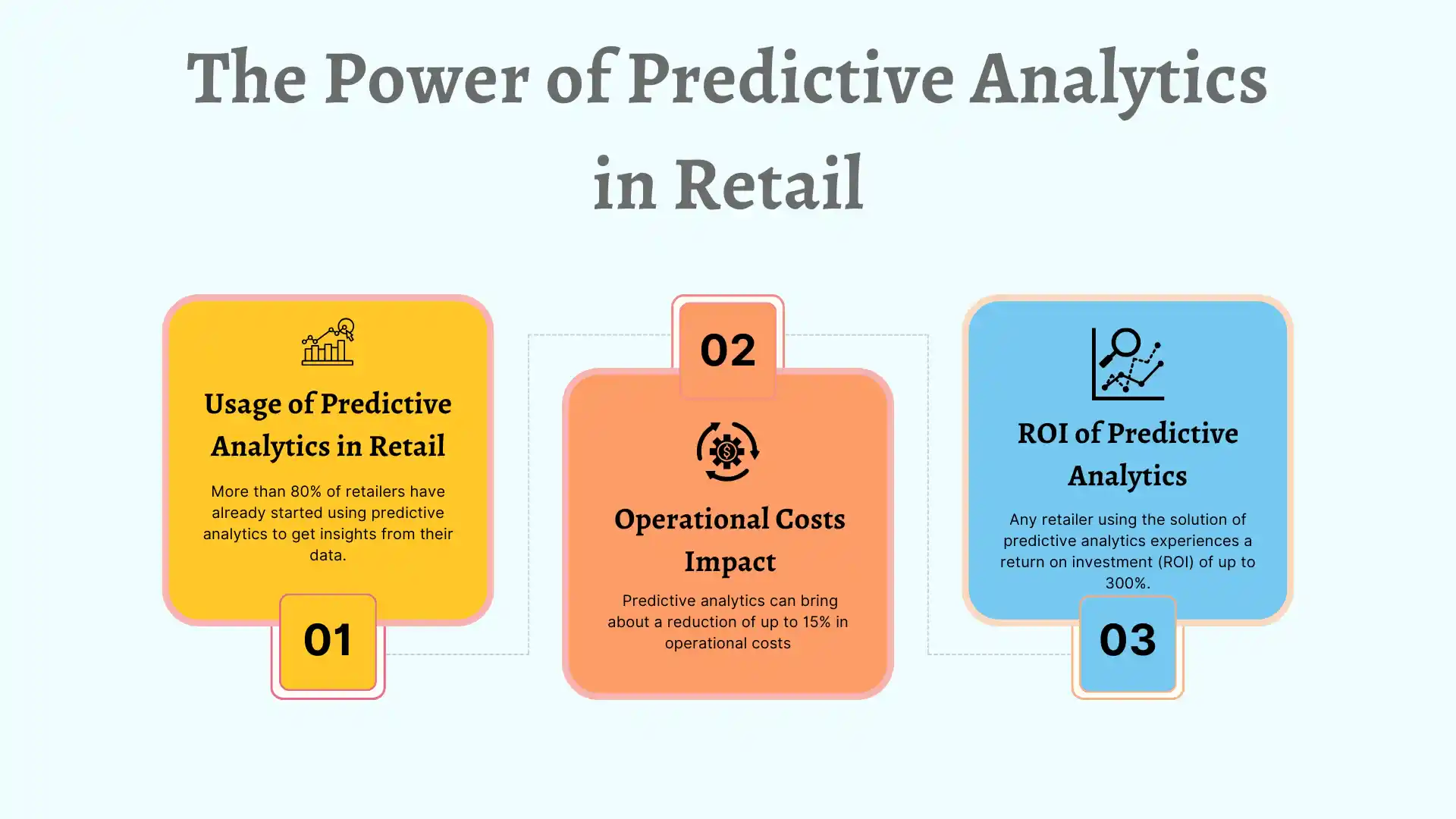
10 Ways to Improve Retail Using Predictive Analytics
Predictive technology is no longer just a trend, but it’s a transforming tool for business that helps organizations anticipate the needs of customers, optimize their operations, and ensure growth. Here are ten ways through which predictive technologies can help improve retailing:
- Customized Customer Experience
Predictive Analytics can enable the customization of shopping experiences for retailers’ customers based on observed behavior and purchase patterns. This could mean recommending items to purchase based on previous buys or offering customized discounts. Business thus goes a long way in satisfying customers and getting more conversions. - Demand Forecasting and Inventory Management
Accurate prediction of customer demand by retailers helps them optimize their stock levels. Predictive models can predict seasonal trends, special promotions, or unexpected surges in demand, thus preventing stockouts or overstocking. This brings about a better inventory turnover ratio and reduced operational costs. - Dynamic Pricing Optimization
Predictive Analytics can therefore change the price for any retail business in real time given prevailing market conditions. For example, dynamic pricing ensures a profit margin of 3-5 % among organizations in capturing higher value at more demanding times or selling cheaper to cover less demanding times. - Improving Customer Retention
Predictive models identify at-risk churning customers, so retailers can target interventions at these groups. A key research finding is that customer retention is vastly cheaper than customer acquisition—studies estimate that increasing customer retention by 5% can boost profits by 25% to 95%. - Optimization of Supply Chain Efficiency
Predictive models improves the supply chain by anticipating delays stockouts and possible disruptors. It can analyze historical data as well as external factors such as weather or geopolitical events. This can enable retailers to make better decisions on when and how to stock their shelves. - Fraud Detection and Prevention
In addition to identifying fraudulent activities early, retailers can prevent fraudulent activity from escalating by using predictive technology. Businesses can identify unusual activity in transactions and customers’ behavior by analyzing patterns, thus preventing financial losses and improving security. - Enhanced Marketing Strategies
This can help retailers identify the most effective marketing strategies and allocate their budget according to that. Be it social media, email marketing, or promotions, businesses can optimize their campaigns to reach the right audience with the right message, which means a boost in ROI. - Store Layout and Merchandising Optimization
Retailers may plan layouts according to foot traffic and consumer needs. For example, if they can predict the products most in demand, the layouts can be rearranged such that the items in demand are more visible, leading to more sales. Predictive models can increase sales by up to 10-15% as product placements better coincide with customer buying patterns - Real-time Sales Monitoring and Performance Measurement
Tracking sales in real-time can help retailers make adjustments to strategies. The real-time data allows businesses to find out which products or areas are not performing and make corrections in time to boost revenue by as much as 10%. - Employee Scheduling and Labour Optimization
Retailers can use predictive models to optimize staffing levels by forecasting busy times based on historical data. This means that stores are adequately staffed during peak hours and not overstaffed when business is slow.
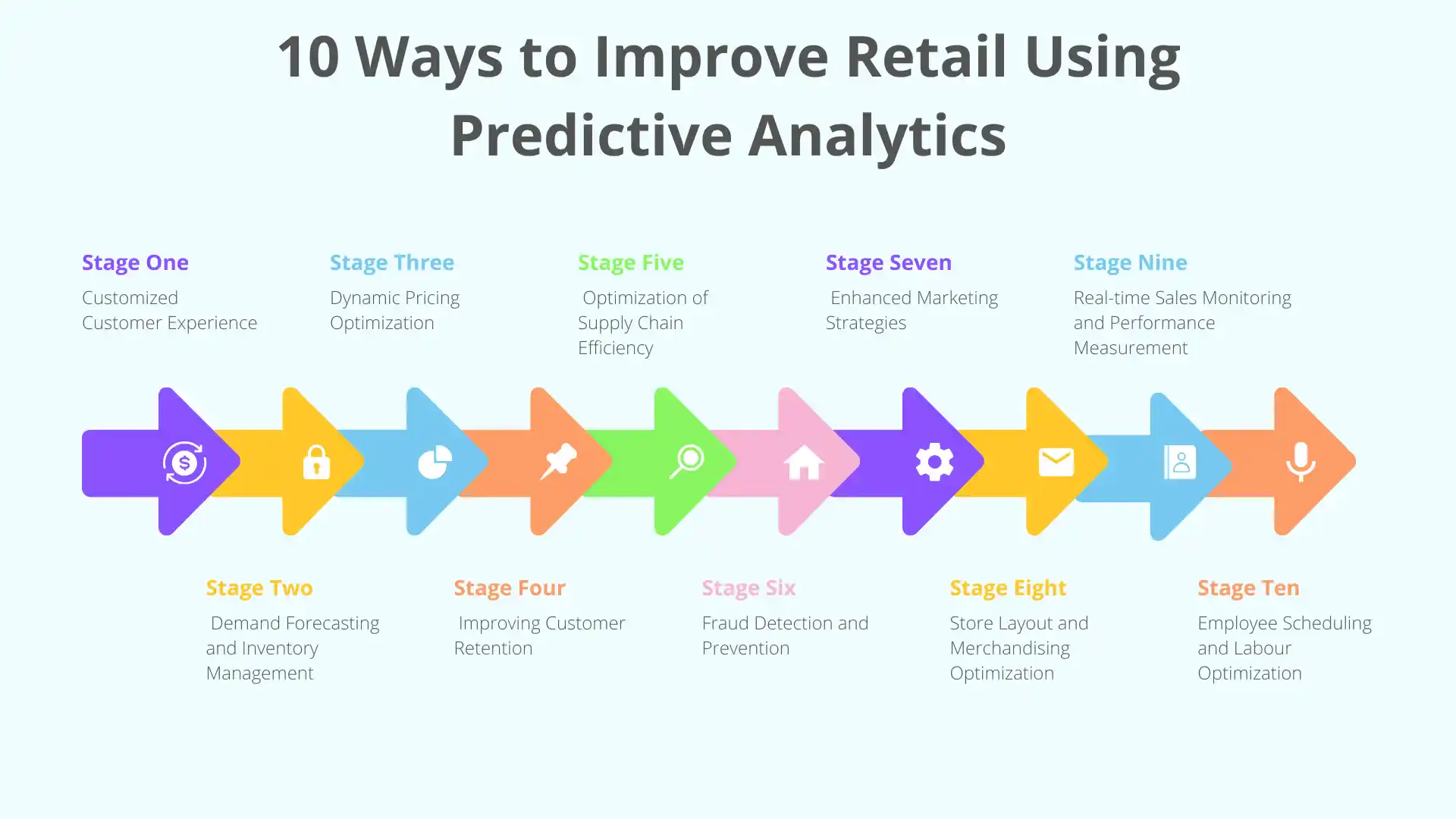
Overcoming Barriers to Predictive Analytics in Retail: Solutions and Challenges
Even though predictive analytics has great potential in the retail space, there are quite several challenges that restrict its use and implementation. Below are the numerous barriers and the innovative solutions on how to overcome them:
- Data Silos and Fragmentation
Challenge: Many retail companies find difficulty building a single comprehensive view of the users and the customers since they are always scattered across many systems.
Solution: Set up a centralized data warehouse and/or collection of cloud-based platforms like Snowflake or AWS that integrate heterogeneous data sources within a complete dataset for analysis. - Lack of Skilled Talent
Challenge: There is a shortage of data scientists and analysts who can handle predictive modeling and be able to use other AI tools in the retail industry.
Solution: Train up the internal team instead, using people who have skills in things like Tableau and Power BI. This gives an outlet to internalize some workforce functionalities or bring in third-party talent and/or consultancies to fill the talent gaps. - High Implementation Costs
Challenge: For small and medium-sized retailers, developing predictive technology systems to support their business objectives becomes just way too pricey.
Solution: Use scalable solutions e.g. predictive offerings delivered on a SaaS basis that require a much lower up-front commitment and are scaled as the need increases. - Data Privacy Concerns
Challenge: Using customer data for analysis poses a problem of compliance with laws like GDPR and CCPA.
Solution: Cost-effective investments in strong anonymization solutions, as well as built-into privacy compliance tools like OneTrust, are recommended for retailers.
Conclusion: Engage Predictive Analytics for Success in Retail
Predictive technologies have changed the face of retail, pushing enterprises to rely less and less on guesswork and more on real actions with data. Examples of these kinds of data-driven activities include, optimizing inventories, personalizing marketing, forecasting demand, improving the customer experience, and many more. All these applications have a lot of potential and great impact. However, there would be some data silos, integration complexity, and even privacy-related issues that might affect the predictive analytics projects. Nevertheless, deploying the right tools and strategies can put retailers through the dilemma and open those floodgates of immense value.
Let’s step into the future of retail with the power of data. Embrace predictive analytics and turn today’s insights into tomorrow’s competitive edge!