Table of Contents
In the ever-advancing domain of artificial intelligence (AI), the imperative for transparency and trust in decision-making processes has become more pronounced. Addressing this imperative head-on is Explainable AI (xAI), a crucial player in demystifying the intricacies of AI systems.
In this article, we embark on a journey through the transformative landscape of AI, exploring how its evolution contributes significantly to the cultivation of trust in AI. As AI technologies permeate diverse facets of our lives, the opaqueness of their decision-making has spurred skepticism and apprehension.
Enter xAI, designed to unravel the complexity of AI algorithms and provide clear insights into their workings. This transparency not only enhances the interpretability of AI but also establishes a foundation for trust.
By dissecting the advancements in AI, we aim to shed light on the mechanisms that empower users to comprehend AI decisions, ultimately fostering a sense of assurance and reliability in the capabilities of artificial intelligence. Join us as we navigate the nuanced terrain of AI and its profound impact on reshaping the narrative around trust in AI systems.
Understanding xAI
Explainable AI (xAI) stands at the forefront of a transformative era in artificial intelligence, signifying a departure from opaque, black-box models. Unlike traditional AI systems that operate as enigmatic decision-making entities, XAI is engineered to illuminate the decision-making processes of AI systems.
This paradigm shift addresses a fundamental challenge in the AI landscape—lack of transparency. By offering clear insights into the rationale behind specific decisions, AI enhances the interpretability of AI models, making them more accessible to users. The significance of AI extends beyond technical intricacies; it encompasses the establishment of trust between users and AI systems.
In a world where skepticism surrounds the workings of advanced algorithms, xAI becomes a beacon of transparency. Users can now understand not only the outcomes produced by AI but also the logic and factors considered in arriving at those outcomes.
This newfound clarity is foundational in fostering trust, as users gain visibility into the decision-making mechanisms that impact their interactions with AI. In essence, AI represents a critical step towards demystifying the complex domain of artificial intelligence, laying the groundwork for a more inclusive, understandable, and trustworthy AI landscape.
The Evolution of xAI
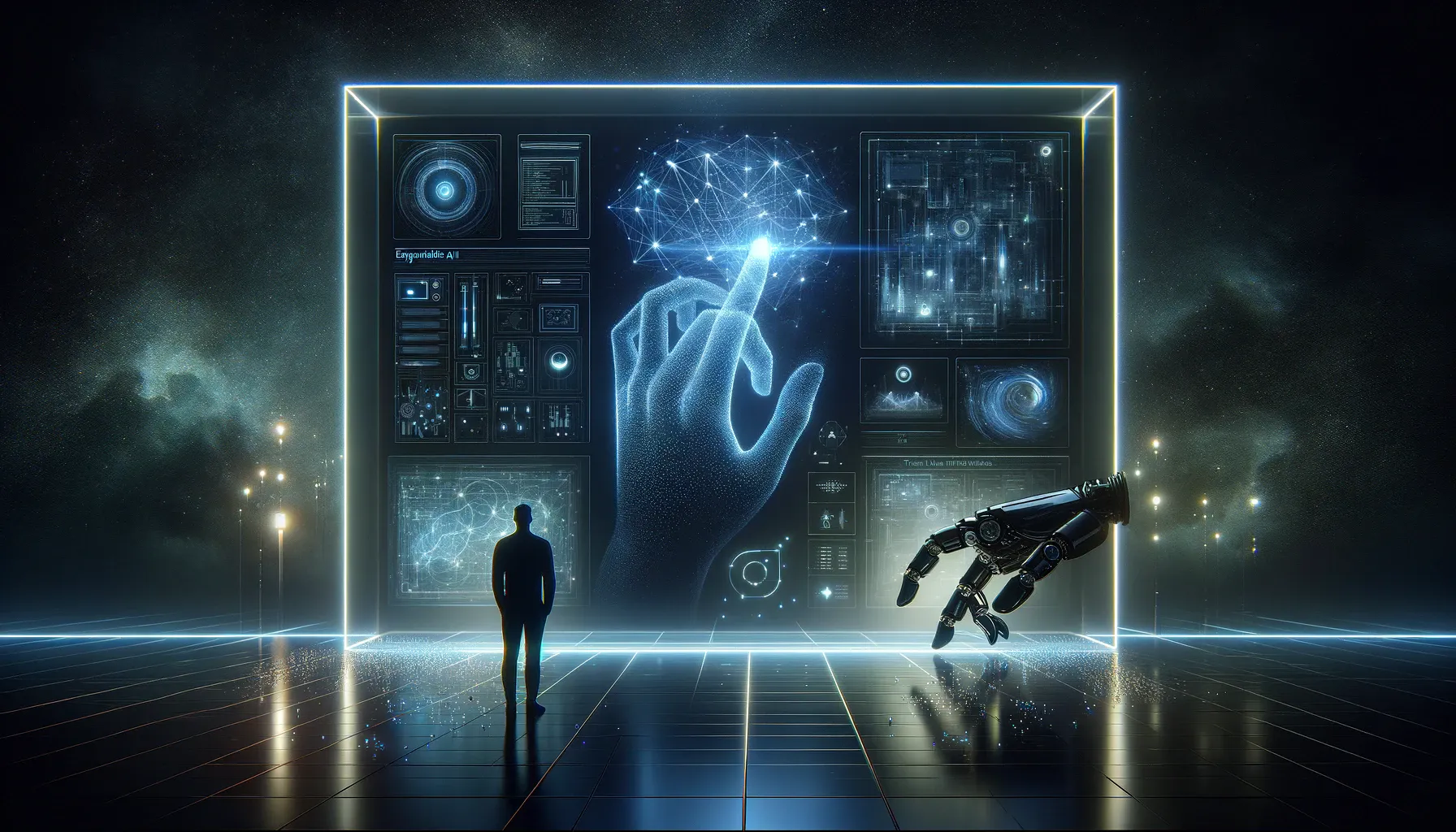
Over time, Explainable AI (xAI) has evolved significantly to address the opacity that characterized early AI models. Initially, users were often left uninformed about decision-making processes, causing skepticism. The advent of AI marked a shift toward models that not only excel in accuracy but also provide transparent rationales for decisions.
Various techniques have emerged, including rule-based systems, ensuring decisions align with predefined rules; model-agnostic interpretability, offering transparency across diverse AI models; and post-hoc interpretability, explaining decisions after they occur.
Real-world applications showcase the success of xAI, such as in finance, where rule-based systems ensure compliance and user trust. In healthcare, model-agnostic interpretability aids in comprehending complex medical decisions, fostering confidence.
These advancements underscore how xAI techniques enhance transparency, mitigate skepticism, and positively impact user trust in AI systems.
Enhanced User Understanding
Explainable AI (xAI) stands as a beacon of transparency, bridging the gap between intricate AI models and non-technical users. In finance, xAI ensures financial decisions are comprehensible, enabling users to grasp risk assessments and investment strategies.
Healthcare benefits from xAI by providing interpretable insights into diagnostic and treatment recommendations, empowering medical professionals and patients. Autonomous vehicles, reliant on complex algorithms, use AI to communicate decision rationale to passengers, fostering trust in the vehicle’s actions.
These instances exemplify how AI enhances user understanding across diverse domains, facilitating collaborative synergy between individuals and AI systems. By demystifying the once-opaque nature of AI, xAI not only ensures effective collaboration but also cultivates a sense of trust, crucial for the widespread acceptance and integration of AI technologies in everyday life.
Addressing Bias and Fairness
Trust in AI hinges on its ability to operate fairly and without bias. Explainable AI (xAI) addresses this crucial concern by actively identifying and mitigating biases in AI algorithms. Advancements in xAI contribute significantly to fostering more equitable AI systems, thereby diminishing the potential impact of biased decision-making.
Case studies and research findings underscore the success of AI in detecting and rectifying bias across diverse AI applications. For instance, in financial services, xAI tools have been implemented to uncover and eliminate biases in credit scoring algorithms, ensuring fair lending practices.
In healthcare, xAI has played a key role in identifying and addressing biases in predictive models, contributing to more accurate and unbiased patient care recommendations. The transparency offered by xAI is paramount in holding AI systems accountable for their decisions.
Users and stakeholders can gain insights into the decision-making processes, understand the factors influencing outcomes, and verify the absence of discriminatory elements. This transparency not only builds trust but also establishes a framework for continuous improvement, as developers can fine-tune models based on feedback and ensure fairness in AI applications.
Industry Applications
In the finance sector, xAI has become integral for regulatory compliance, ensuring transparency and interpretability in complex models. Institutions like JPMorgan Chase and Goldman Sachs have successfully adopted AI, enhancing trust by providing clear explanations for algorithmic decisions.
This not only aids compliance but also strengthens relationships with clients and regulators. In healthcare, where trust is paramount, xAI plays a crucial role. Companies like IBM Watson Health and Siemens Healthineers leverage AI to make AI-driven medical decisions more understandable.
This transparency builds trust among healthcare professionals and patients, promoting the widespread adoption of AI technologies in diagnostic and treatment processes. Noteworthy success stories include Google’s use of AI in its search algorithms, and improving user satisfaction by explaining personalized search results.
Additionally, companies like ZestFinance employ xAI in credit scoring models, increasing transparency and trust in lending decisions. These samples underscore how AI not only addresses industry-specific challenges but also enhances user confidence, fostering positive relationships and facilitating broader acceptance of AI technologies across diverse sectors.
Challenges and Future Outlook
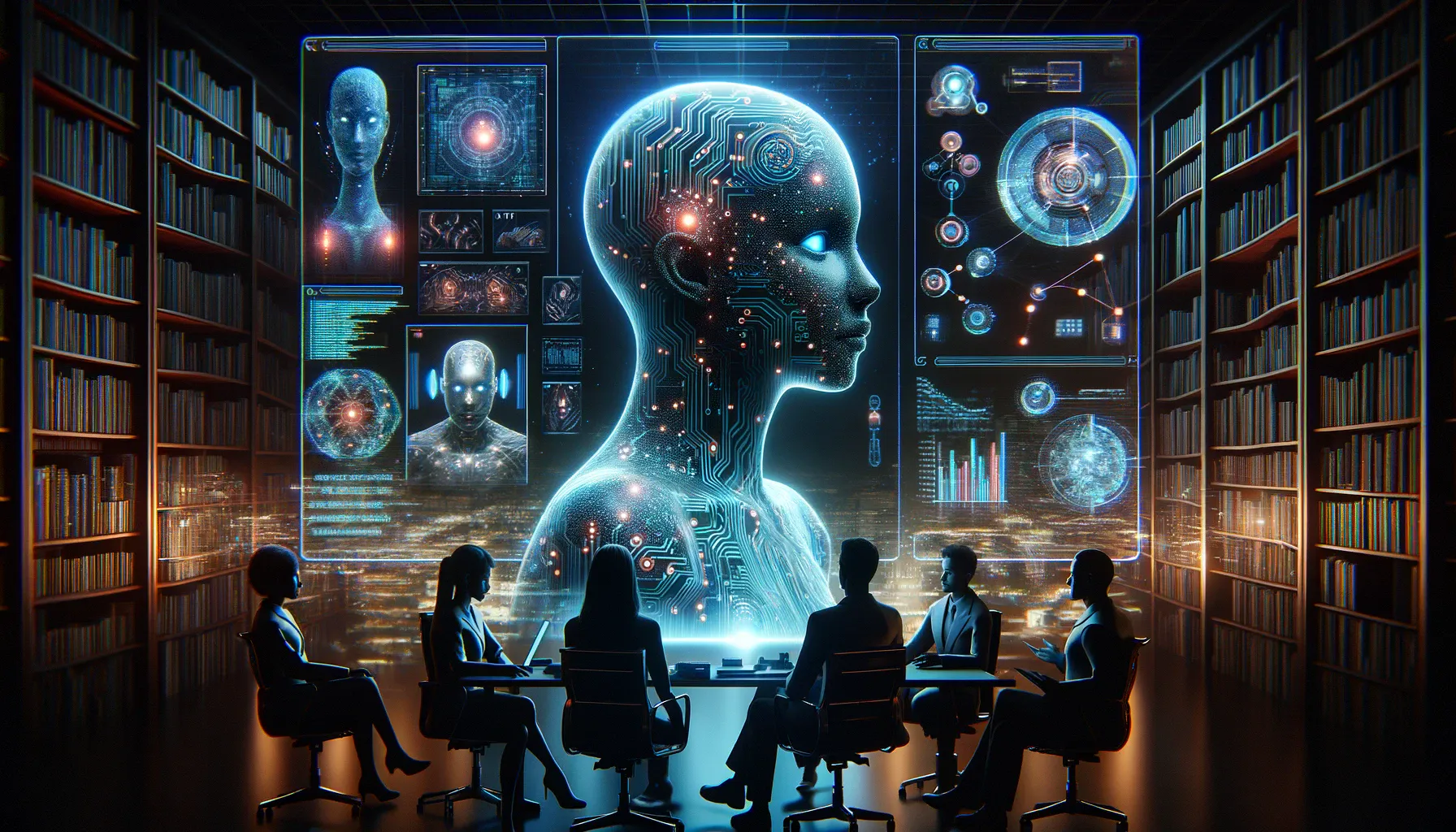
Despite the strides made by xAI in enhancing transparency, challenges persist. Ongoing research is dedicated to overcoming these hurdles and pushing the boundaries of explainable AI. Scholars and practitioners are actively working on refining interpretability techniques to ensure that AI decision-making processes remain accessible and understandable.
The focus extends to developing models that not only provide explanations but also adapt to diverse and dynamic datasets. Ethical considerations loom large in the AI landscape. Striking a delicate balance between transparency and safeguarding sensitive information is paramount.
Developers are grappling with the ethical implications of revealing too much information, especially in contexts where privacy is a concern. Efforts are directed towards creating mechanisms that offer transparency without compromising individual privacy rights. The importance of continuous research and collaboration cannot be overstated.
A collaborative approach involving researchers, industry experts, and policymakers is crucial to navigating the complex ethical and technical challenges associated with AI. Ongoing advancements hinge on shared knowledge, interdisciplinary collaboration, and a commitment to refining xAI techniques.
By fostering an environment of collective learning and innovation, the future of xAI holds promise in overcoming challenges and further establishing itself as a cornerstone for trustworthy and ethical AI systems.
Case Studies
The renowned Mercy Medical Center adopts an xAI-driven diagnostic system for medical imaging, enhancing transparency. Interpretability builds trust as models provide clear explanations for diagnoses, boosting acceptance and confidence among both healthcare professionals and patients.
Incorporating xAI, Smith & Associates law firm enhances legal predictions. Transparent insights into decision factors empower lawyers, fostering trust with clients. The xAI system, with its accuracy, establishes Smith & Associates as a trusted authority in the legal community.
An automotive giant like Tesla integrates xAI into its autonomous vehicles, providing clear insights into navigation, obstacle avoidance, and emergency responses. This case study illuminates how Tesla’s transparent AI systems enhance public trust, alleviating concerns about safety and reliability in self-driving cars.
Horizon Banking, a global financial institution, integrates xAI, like FraudGuard, into its fraud detection system, utilizing rule-based models and feature importance analysis. This transparency not only uncovers decision-making in fraud alerts but also enhances trust with customers, regulators, and stakeholders, elevating fraud detection and prevention.
Conclusion
In summary, the strides made in Explainable AI (xAI) play a pivotal role in cultivating trust within the domain of artificial intelligence. The multifaceted impact of xAI is evident in its ability to enhance user understanding, effectively bridging the gap between complex AI processes and end-users.
By offering transparent insights into decision-making mechanisms, xAI not only demystifies intricate algorithms but also empowers individuals to comprehend and trust the outputs generated by AI systems. Moreover, xAI serves as a potent tool in addressing biases inherent in AI models, contributing to the creation of fair and equitable systems.
The transparency it provides allows for the identification and rectification of biases, ensuring that AI systems operate ethically and without discriminatory tendencies. As industries increasingly acknowledge the inherent value of explainability, the future of xAI appears promising.
The ongoing evolution of xAI techniques, coupled with the growing recognition of its importance, suggests a trajectory towards even more sophisticated and transparent AI systems. This continued advancement not only fortifies trust in AI but also propels the technology into domains of application where reliability and interpretability are paramount, solidifying xAI’s role as a cornerstone for trustworthy and ethically sound artificial intelligence systems.